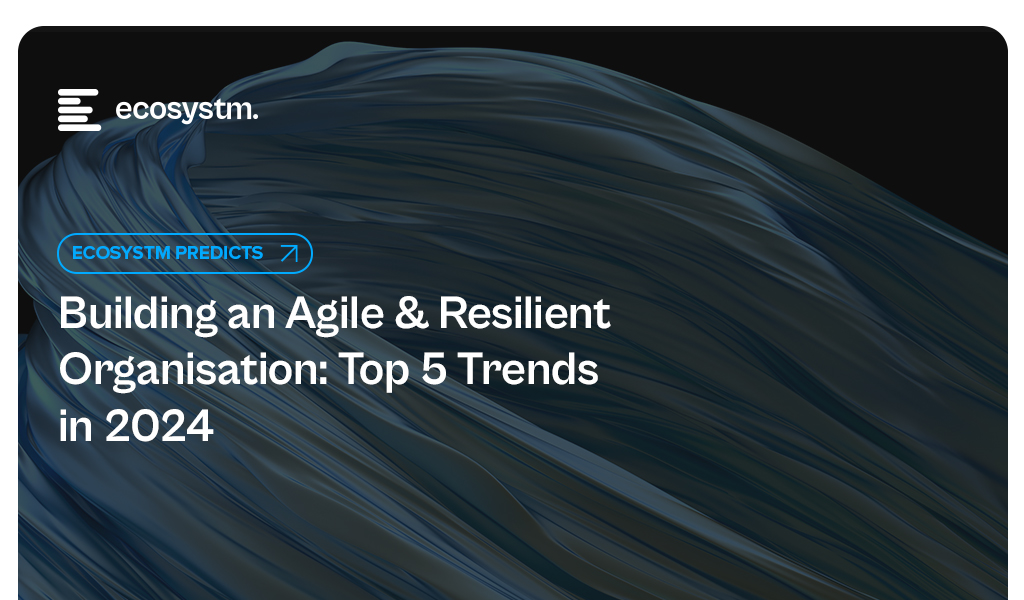
While the discussions have centred around AI, particularly Generative AI in 2023, the influence of AI innovations is extensive. Organisations will urgently need to re-examine their risk strategies, particularly in cyber and resilience practices. They will also reassess their infrastructure needs, optimise applications for AI, and re-evaluate their skills requirements.
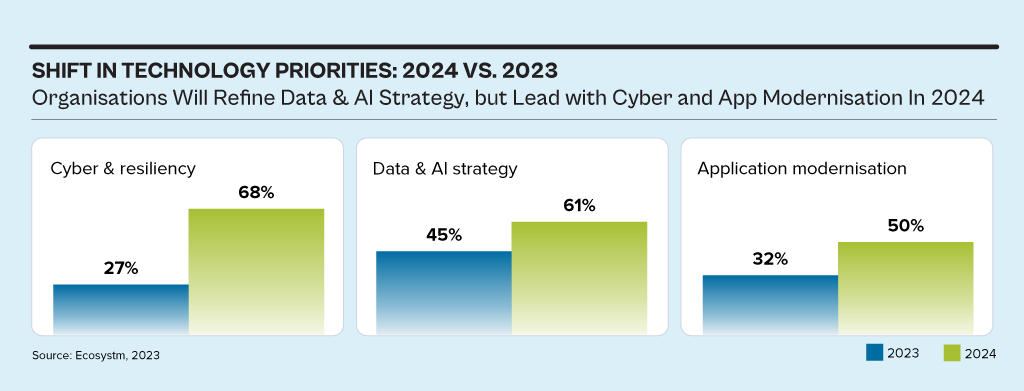
This impacts the entire tech market, including tech skills, market opportunities, and innovations.
Ecosystm analysts Alea Fairchild, Darian Bird, Richard Wilkins, and Tim Sheedy present the top 5 trends in building an Agile & Resilient Organisation in 2024.
Click here to download ‘Ecosystm Predicts: Top 5 Resilience Trends in 2024’ as a PDF.
#1 Gen AI Will See Spike in Infrastructure Innovation
Enterprises considering the adoption of Generative AI are evaluating cloud-based solutions versus on-premises solutions. Cloud-based options present an advantage in terms of simplified integration, but raise concerns over the management of training data, potentially resulting in AI-generated hallucinations. On-premises alternatives offer enhanced control and data security but encounter obstacles due to the unexpectedly high demands of GPU computing needed for inferencing, impeding widespread implementation. To overcome this, there’s a need for hardware innovation to meet Generative AI demands, ensuring scalable on-premises deployments.
The collaboration between hardware development and AI innovation is crucial to unleash the full potential of Generative AI and drive enterprise adoption in the AI ecosystem.
Striking the right balance between cloud-based flexibility and on-premises control is pivotal, with considerations like data control, privacy, scalability, compliance, and operational requirements.
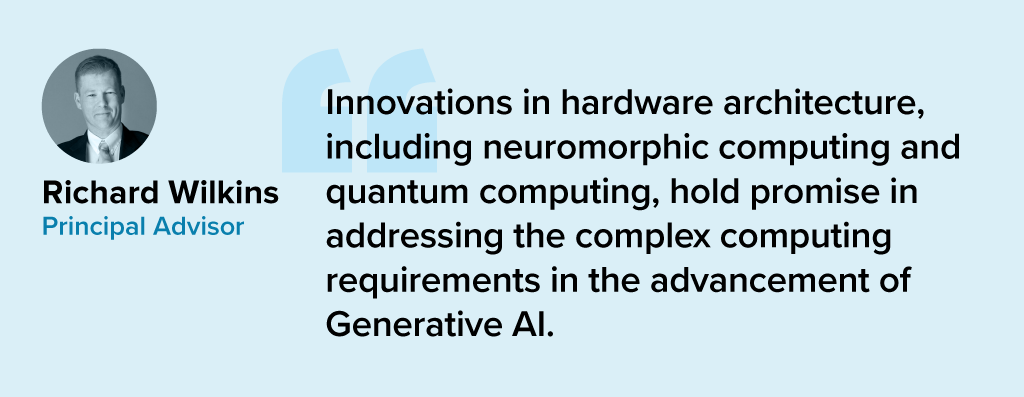
#2 Cloud Migrations Will Make Way for Cloud Transformations
The steady move to the public cloud has slowed down. Organisations – particularly those in mature economies – now prioritise cloud efficiencies, having largely completed most of their application migration. The “easy” workloads have moved to the cloud – either through lift-and-shift, SaaS, or simple replatforming.
New skills will be needed as organisations adopt public and hybrid cloud for their entire application and workload portfolio.
- Cloud-native development frameworks like Spring Boot and ASP.NET Core make it easier to develop cloud-native applications
- Cloud-native databases like MongoDB and Cassandra are designed for the cloud and offer scalability, performance, and reliability
- Cloud-native storage like Snowflake, Amazon S3 and Google Cloud Storage provides secure and scalable storage
- Cloud-native messaging like Amazon SNS and Google Cloud Pub/Sub provide reliable and scalable communication between different parts of the cloud-native application
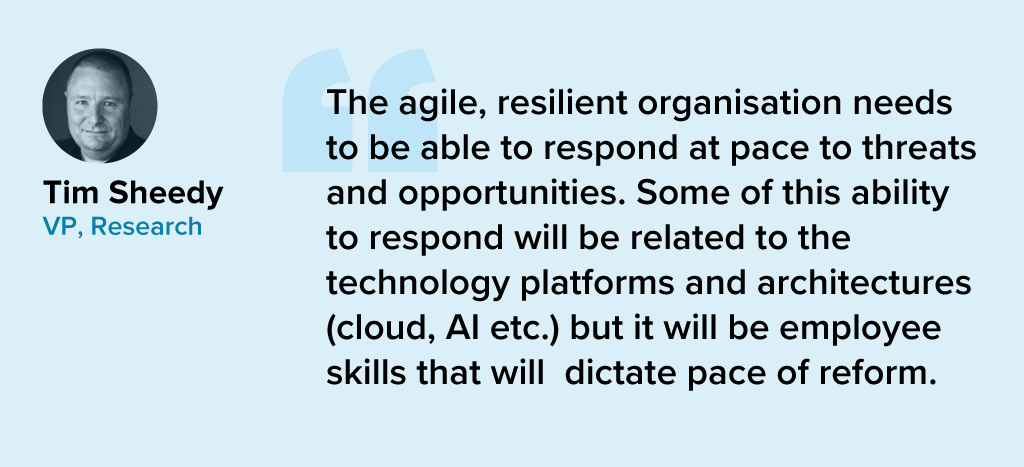
#3 2024 Will be a Good Year for Technology Services Providers
Several changes are set to fuel the growth of tech services providers (systems integrators, consultants, and managed services providers).
There will be a return of “big apps” projects in 2024.
Companies are embarking on significant updates for their SAP, Oracle, and other large ERP, CRM, SCM, and HRM platforms. Whether moving to the cloud or staying on-premises, these upgrades will generate substantial activity for tech services providers.
The migration of complex apps to the cloud involves significant refactoring and rearchitecting, presenting substantial opportunities for managed services providers to transform and modernise these applications beyond traditional “lift-and-shift” activities.
The dynamic tech landscape, marked by AI growth, evolving security threats, and constant releases of new cloud services, has led to a shortage of modern tech skills. Despite a more relaxed job market, organisations will increasingly turn to their tech services partners, whether onshore or offshore, to fill crucial skill gaps.
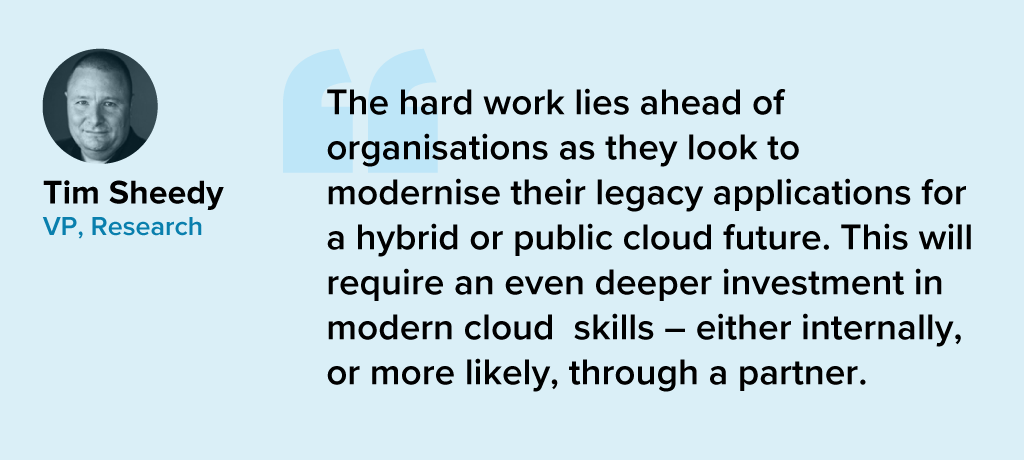
#4 Gen AI and Maturing Deepfakes Will Democratise Phishing
As with any emerging technology, malicious actors will be among the fastest to exploit Generative AI for their own purposes. The most immediate application will be employing widely available LLMs to generate convincing text and images for their phishing schemes. For many potential victims, misspellings and strangely worded appeals are the only hints that an email from their bank, courier, or colleague is not what it seems. The ability to create professional-sounding prose in any language and a variety of tones will unfortunately democratise phishing.
The emergence of Generative AI combined with the maturing of deepfake technology will make it possible for malicious agents to create personalised voice and video attacks. Digital channels for communication and entertainment will be stretched to differentiate between real and fake.
Security training that underscores the threat of more polished and personalised phishing is a must.
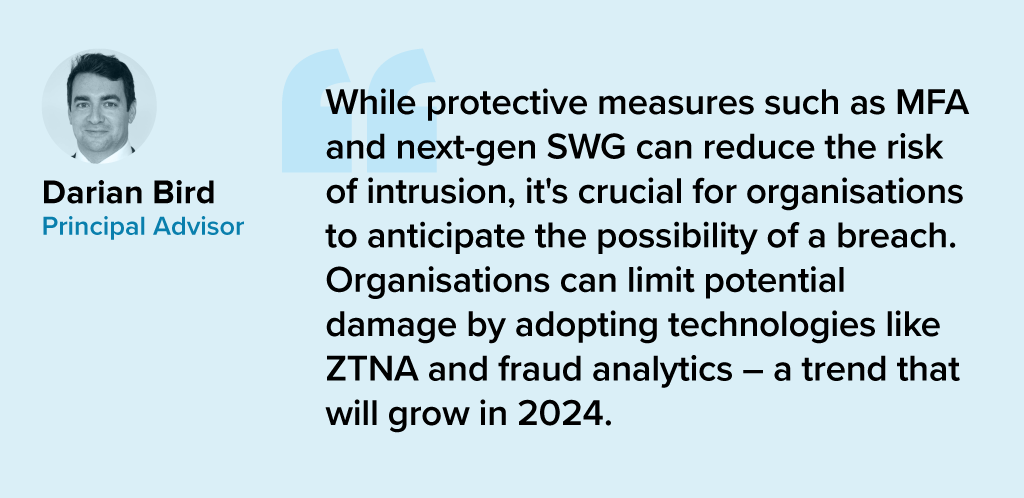
#5 A Holistic Approach to Risk and Operational Resilience Will Drive Adoption of VMaaS
Vulnerability management is a continuous, proactive approach to managing system security. It not only involves vulnerability assessments but also includes developing and implementing strategies to address these vulnerabilities. This is where Vulnerability Management Platforms (VMPs) become table stakes for small and medium enterprises (SMEs) as they are often perceived as “easier targets” by cybercriminals due to potentially lesser investments in security measures.
Vulnerability Management as a Service (VMaaS) – a third-party service that manages and controls threats to automate vulnerability response to remediate faster – can improve the asset cybersecurity management and let SMEs focus on their core activities.
In-house security teams will particularly value the flexibility and customisation of dashboards and reports that give them enhanced visibility over all assets and vulnerabilities.
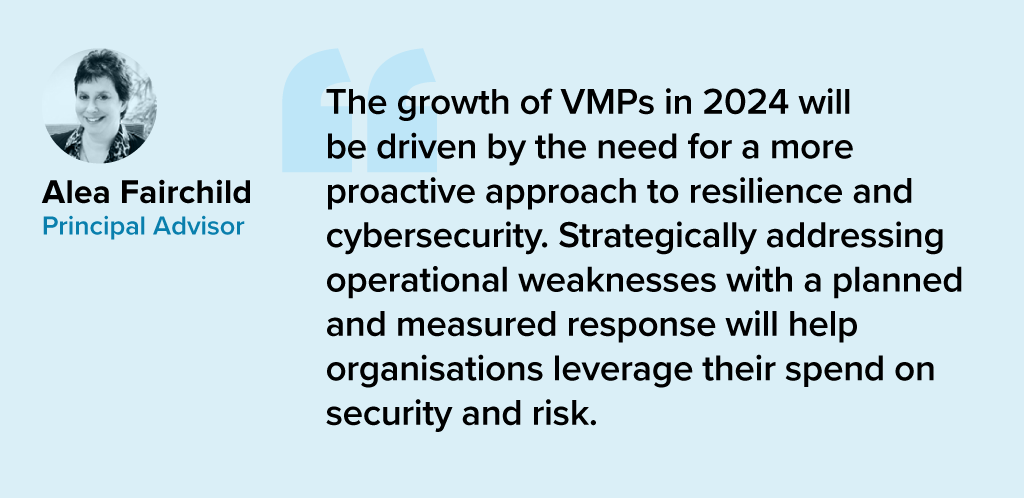
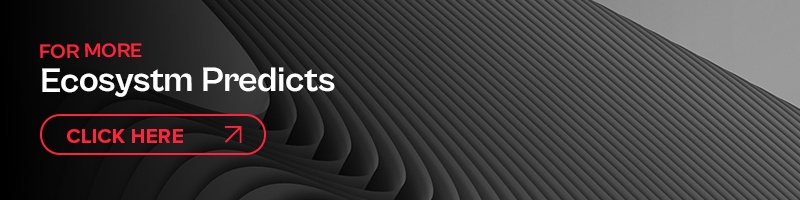
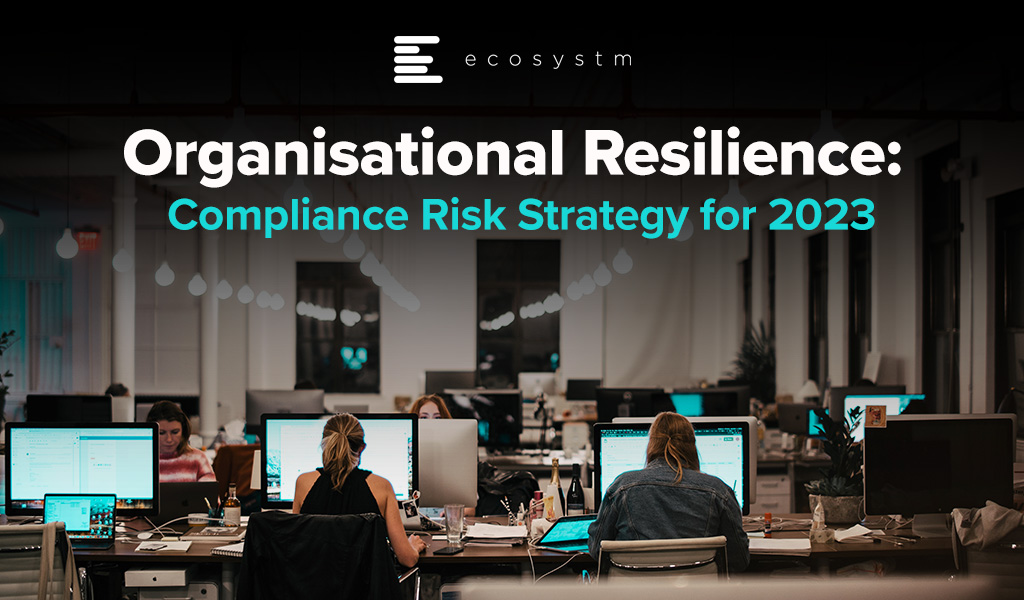
There are a number of updates to regulations that will impact organisations in 2023. They will create new requirements for businesses to follow, new areas of risk, and more money and time spent adjusting to these changes.
Compliance strategies help cement trust in professional partnerships and vendor relationships. Whether organisations are trying to qualify for cyber insurance, or simply looking to obey the law and avoid fines, they are up against increasingly tough compliance measures. It is no longer sufficient to be compliant only once in a year, scramble in the two weeks before the audit, and then forget about it for the rest of the year.
What compliance tech trends should IT management adopt as they build and refine their technology roadmaps?
Let’s look at some regulatory and technology trends.
Regulations to Watch
European Union Digital Operational Resilience Act (DORA). The EU is applying regulatory pressure on the financial services industry with its Digital Operational Resilience Act (DORA). DORA is a “game changer” that will push firms to fully understand how their IT, operational resilience, cyber and third-party risk management practices affect the resilience of their most critical functions as well as develop entirely new operational resilience capabilities.
One key element that DORA introduces is the Critical Third Party (CTP) oversight framework, expanding the scope of the financial services regulatory perimeter and granting the European Supervisory Authorities (ESAs) substantial new powers to supervise CTPs and address resilience risks they might pose to the sector.
Germany’s Supply Chain Due Diligence Act (SCDDA). On January 1, 2023, the Supply Chain Due Diligence Act took effect. It requires all companies with head offices, principal places of business, or administrative headquarters in Germany – with more than 3,000 employees in the country – to comply with core human rights and certain environmental provisions in their supply chains. SCDDA is far-reaching and impacts multiple facets of the supply chain, from human rights to sustainability, and legal accountability throughout the third-party ecosystem. It will address foundational supply chain issues like anti-bribery and corruption diligence.
From 2024, the number of employees will be lowered from 3,000 to 1,000. And Switzerland, The Netherlands, and the European Union also have similar drafts of regulation in the books.
PCI DSS 4.0. Payment Card Industry Data Security Standard (PCI DSS) is the core component of any credit card company’s security protocol. In an increasingly cashless world, card fraud is a growing concern. Any company that accepts, transmits, or stores a cardholder’s private information must be compliant. PCI compliance standards help avoid fraudulent activity and mitigate data breaches by keeping the cardholder’s sensitive financial information secure.
PCI compliance standards require merchants to consistently adhere to the PCI Standards Council’s guidelines which include 78 base requirements, more than 400 test procedures, and 12 key requirements.
Looking at how PCI has evolved over the years up to PCI 4.0, there is a departure from specific technical requirements toward the general concept of overall security. PCI 4.0 requirements were released in March 2022 and will become mandatory in March 2024 for all organisations that process or store cardholder data.
The costs of maintaining compliance controls and security measures are only part of what businesses should consider for PCI certification. Businesses should also account for audit costs, yearly fees, remediation expenses, and employee training costs in their budgets as well as technical upgrades to meet compliance standards.
Tech Trend Changes
Zero Trust presents a shift from a location-centric model to a more data-centric approach for fine-grained security controls between users, systems, data, and assets. Zero Trust as a model assumes all requests are from an open network and verifies each request this way. PCI 4.0 does not mention Zero Trust architecture specifically, but it is evident that the Security Standards Council is going that way as a future consideration.
Passwordless authentication has gained a lot of attention and traction recently. large tech providers such as Google, Apple, and Microsoft, are introducing passwordless authentication based on passkeys. This is a clear sign that the game is about to change. As the PCI DSS focuses on avoiding fraudulent activity, so does newer authentication protocol approaches to verify and confirm identity.
Third-party risk management is quickly evolving into third-party trust management (TPTM), with the SCDDA creating a clear line in the sand for global organisations. TPTM is a critical consideration when standing up an enterprise trust strategy. Enterprise trust is a driver of business development that depends on cross-domain collaboration. It goes beyond cybersecurity and focuses on building trusted and lasting third-party relationships across the core critical risk domains: security, privacy, ethics & compliance, and ESG.
Final thought – Cyber Insurance in 2023
If some of these compliance drivers lead to a desire for financial protection, cyber insurance is one mitigation element for strategy to address C-level concerns. But wait – this is not as easy as it used to be.
Five years ago, a firm could fill out a one-page cyber insurance application and answer a handful of questions. Fast forward to today’s world of ransomware attacks and other cyber threats – now getting insurance with favourable terms, conditions, pricing, coverage and low retention is tough.
Insurance companies prefer enterprises that are instituting robust security controls and incident response plans — especially those prepared to deep dive into their cybersecurity architectures and with planned roadmaps. In terms of compliance strategy development, there needs to be a risk-based approach to cybersecurity to allow an insurer to offer a favourable insurance option.
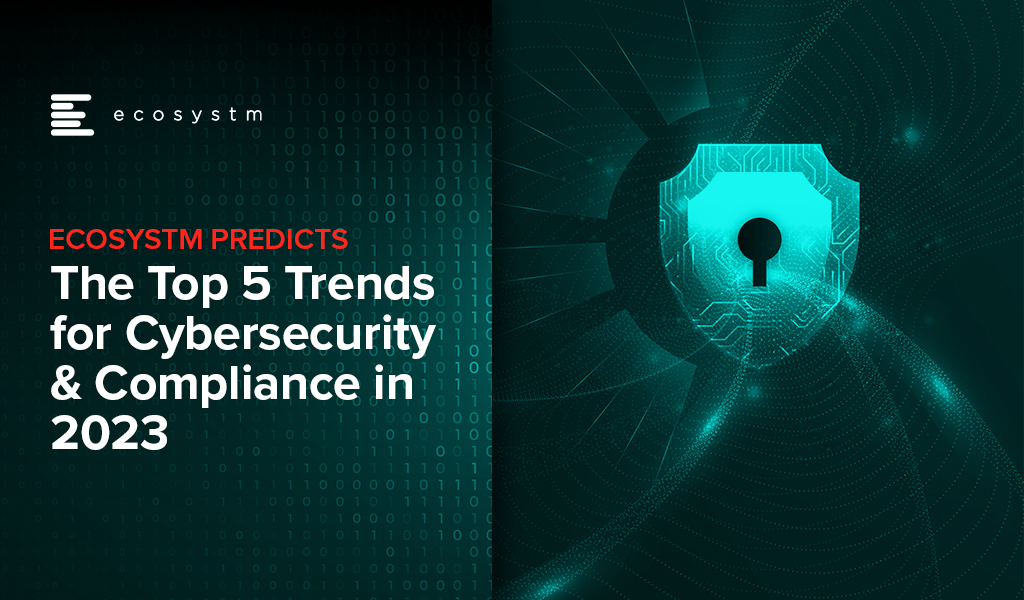
With organisations facing an infrastructure, application, and end-point sprawl, the attack surface continues to grow; as do the number of malicious attacks. Cyber breaches are also becoming exceedingly real for consumers, as they see breaches and leaks in brands and services they interact with regularly. 2023 will see CISOs take charge of their cyber environment – going beyond a checklist.
Here are the top 5 trends for Cybersecurity & Compliance for 2023 according to Ecosystm analysts Alan Hesketh, Alea Fairchild, Andrew Milroy, and Sash Mukherjee.
- An Escalating Cybercrime Flood Will Drive Proactive Protection
- Incident Detection and Response Will Be the Main Focus
- Organisations Will Choose Visibility Over More Cyber Tools
- Regulations Will Increase the Risk of Collecting and Storing Data
- Cyber Risk Will Include a Focus on Enterprise Operational Resilience
Read on for more details.
Download Ecosystm Predicts: The Top 5 Trends for Cybersecurity & Compliance in 2023 as a PDF
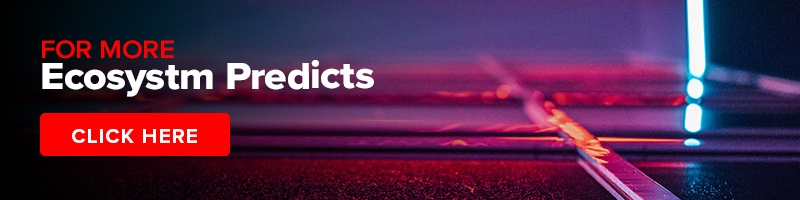
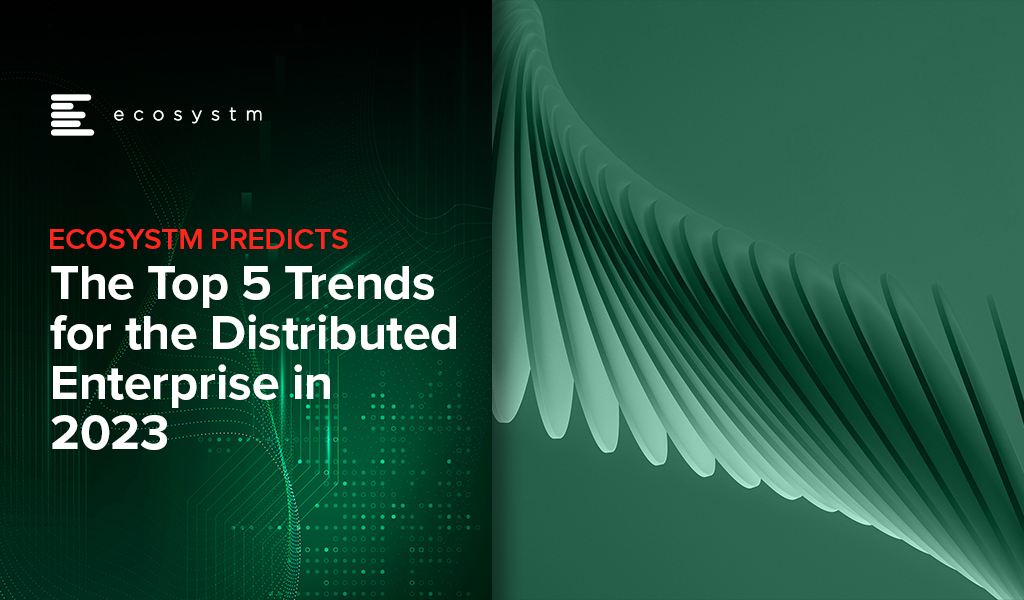
In 2023, organisations will continue to reinvent themselves to remain relevant to their customers, engage their employees and be efficient and profitable.
As per Ecosystm’s Digital Enterprise Study 2022, organisations will increase spend on digital workplace technologies, enterprise software upgrades, mobile applications, infrastructure and data centres, and hybrid cloud management.
Here are the top 5 trends for the Distributed Enterprise in 2023 according to Ecosystm analysts, Alea Fairchild, Darian Bird, Peter Carr, and Tim Sheedy.
- Deskless Workers Will Become Modern Professionals
- Need for Cost Efficiency Will Stimulate the Use of Waste Metrics in Public Cloud
- The Climate & Energy Crisis Will Change the Cloud Equation
- Industry Cloud Will Further Accelerate Business Innovation
- The SASE Piece Will Fall in Place
Read on for more details.
Download Ecosystm Predicts: The Top 5 Trends for the Distributed Enterprise in 2023
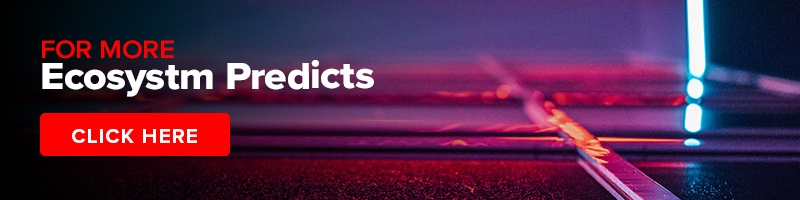
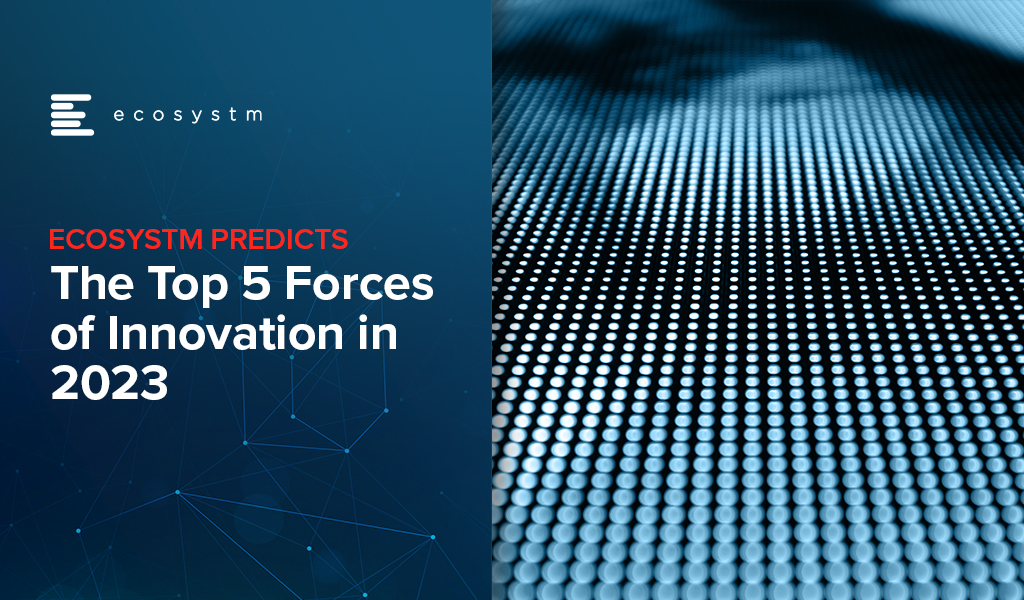
2022 was a year of consolidation – of business strategy, people policy, tech infrastructure, and applications. In 2023, despite the economic uncertainties, organisations will push forward in their tech investments on selected areas, with innovation as their primary focus. Successful businesses today realise that they are operating in a “disrupt or be disrupted” environment.
Here are the top 5 forces of innovation in 2023 according to Ecosystm analysts, Alan Hesketh, Alea Fairchild, Peter Carr, and Tim Sheedy and Ecosystm CEO Ullrich Loeffler.
- The Gen Z Tsunami will force organisations to truly embrace the 21st century.
- “Big Ticket Innovation” will get back on the agenda.
- Over the Edge: The Metaverse ecosystem will take shape.
- Green Computing will drive tech investments.
- Organisations will harness existing tech to innovate.
Read on for more details.
Download Ecosystm Predicts: The Top 5 Forces of Innovation in 2023 as a PDF
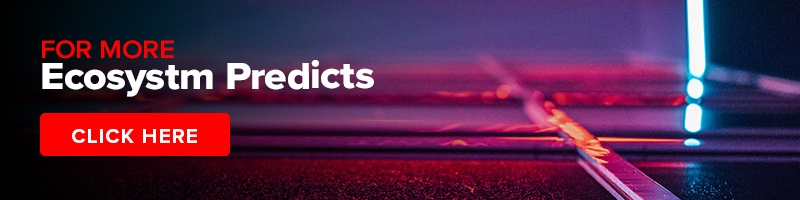
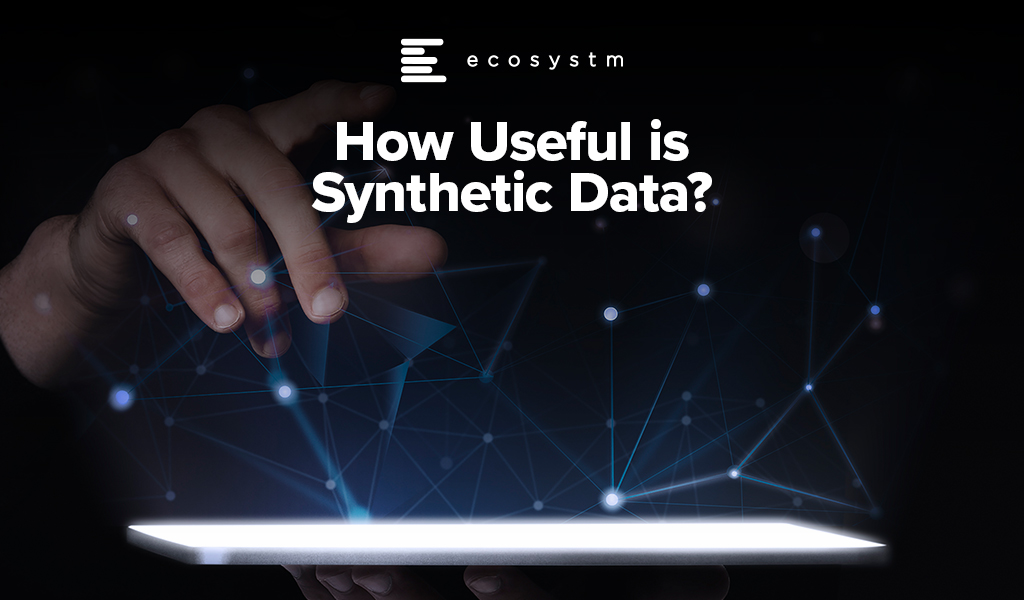
When non-organic (man-made) fabric was introduced into fashion, there were a number of harsh warnings about using polyester and man-made synthetic fibres, including their flammability.
In creating non-organic data sets, should we also be creating warnings on their use and flammability? Let’s look at why synthetic data is used in industries such as Financial Services, Automotive as well as for new product development in Manufacturing.
Synthetic Data Defined
Synthetic data can be defined as data that is artificially developed rather than being generated by actual interactions. It is often created with the help of algorithms and is used for a wide range of activities, including as test data for new products and tools, for model validation, and in AI model training. Synthetic data is a type of data augmentation which involves creating new and representative data.
Why is it used?
The main reasons why synthetic data is used instead of real data are cost, privacy, and testing. Let’s look at more specifics on this:
- Data privacy. When privacy requirements limit data availability or how it can be used. For example, in Financial Services where restrictions around data usage and customer privacy are particularly limiting, companies are starting to use synthetic data to help them identify and eliminate bias in how they treat customers – without contravening data privacy regulations.
- Data availability. When the data needed for testing a product does not exist or is not available to the testers. This is often the case for new releases.
- Data for testing. When training data is needed for machine learning algorithms. However, in many instances, such as in the case of autonomous vehicles, the data is expensive to generate in real life.
- Training across third parties using cloud. When moving private data to cloud infrastructures involves security and compliance risks. Moving synthetic versions of sensitive data to the cloud can enable organisations to share data sets with third parties for training across cloud infrastructures.
- Data cost. Producing synthetic data through a generative model is significantly more cost-effective and efficient than collecting real-world data. With synthetic data, it becomes cheaper and faster to produce new data once the generative model is set up.
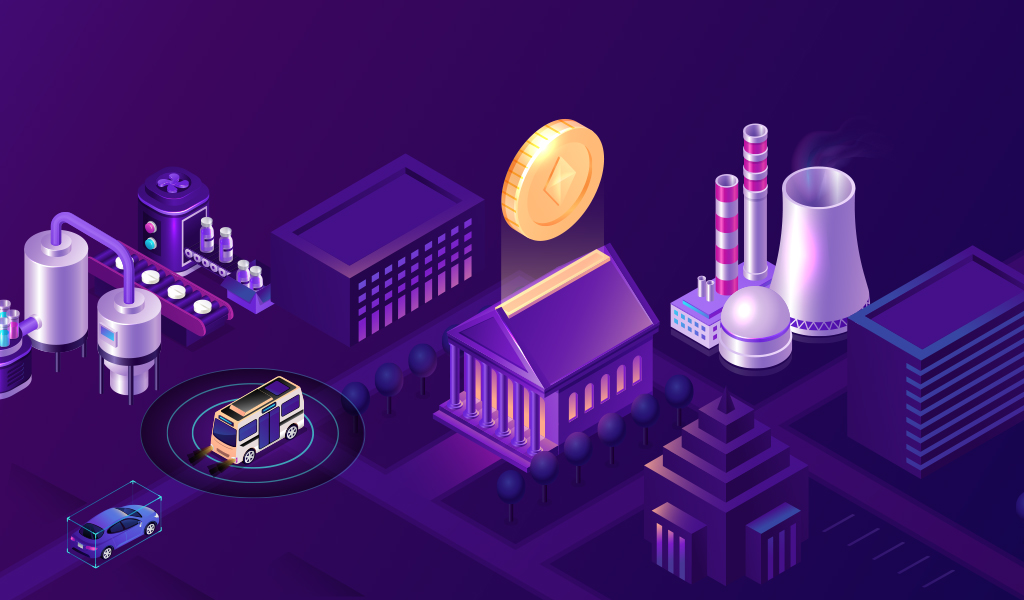
Why should it cause concern?
If real dataset contains biases, data augmented from it will contain biases, too. So, identification of optimal data augmentation strategy is important.
If the synthetic set doesn’t truly represent the original customer data set, it might contain the wrong buying signals regarding what customers are interested in or are inclined to buy.
Synthetic data also requires some form of output/quality control and internal regulation, specifically in highly regulated industries such as the Financial Services.
Creating incorrect synthetic data also can get a company in hot water with external regulators. For example, if a company created a product that harmed someone or didn’t work as advertised, it could lead to substantial financial penalties and, possibly, closer scrutiny in the future.
Conclusion
Synthetic data allows us to continue developing new and innovative products and solutions when the data necessary to do so wouldn’t otherwise be present or available due to volume, data sensitivity or user privacy challenges. Generating synthetic data comes with the flexibility to adjust its nature and environment as and when required in order to improve the performance of the model to create opportunities to check for outliers and extreme conditions.
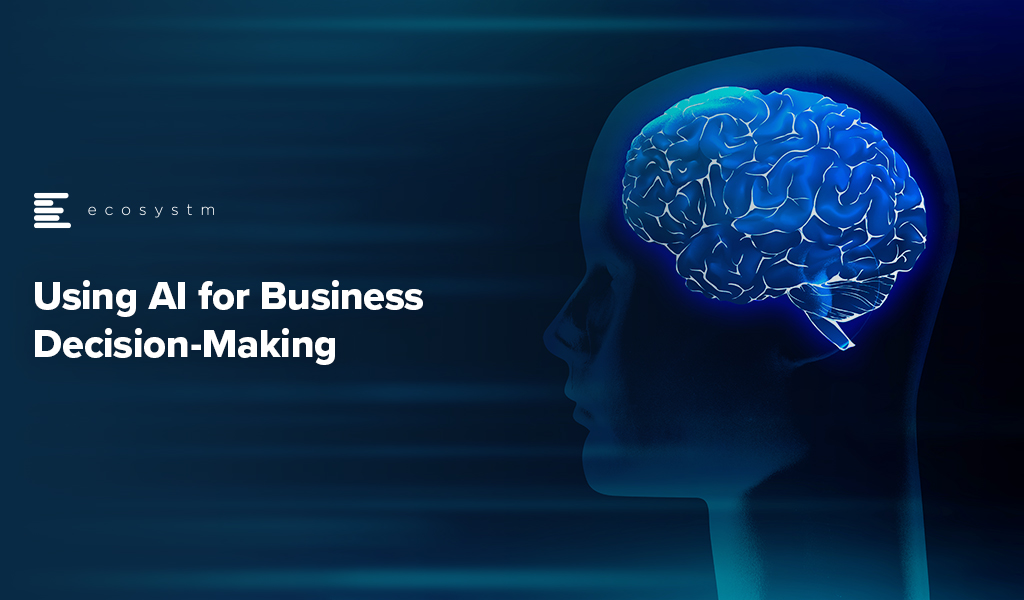
Why do we use AI? The goal of a business in adding intelligence is to enhance business decision-making, and growing revenue and profit within the framework of its business model.
The problem many organisations face is that they understand their own core competence in their own industry, but they do not understand how to tweak and enhance business processes to make the business run better. For example, AI can help transform the way companies run their production lines, enabling greater efficiency by enhancing human capabilities, providing real-time insights, and facilitating design and product innovation. But first, one has to be able to understand and digest the data within the organisation that would allow that to happen.
Ecosystm research shows that AI adoption crosses the gambit of business processes (Figure 1), but not all firms are process optimised to achieve those goals internally.
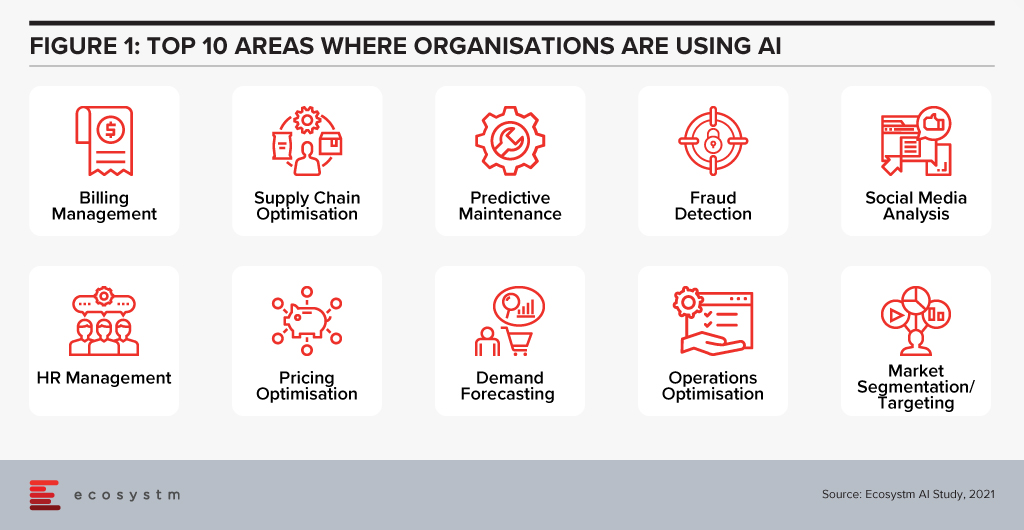
The initial landscape for AI services primarily focused on tech companies building AI products into their own solutions to power their own services. So, the likes of Amazon, Google and Apple were investing in people and processes for their own enhancements.
As the benefits of AI are more relevant in a post-pandemic world with staff and resource shortages, non-tech firms are becoming interested in applying those advantages to their own business processes.
AI for Decisions
Recent start-up ventures in AI are focusing on non-tech companies and offering services to get them to use AI within their own business models. Peak AI says that their technology can help enterprises that work with physical products to make better, AI-based evaluations and decisions, and has recently closed a funding round of USD 21 million.
The relevance of this is around the terminology that Peak AI has introduced. They call what they offer “Decision Intelligence” and are crafting a market space around it. Peak’s basic premise was to build AI not as a business goal for itself but as a business service aided by a solution and limited to particular types of added value. The goal of Peak AI is to identify where Decision Intelligence can add value, and help the company build a business case that is both achievable and commercially viable.
For example, UK hard landscaping manufacturer Marshalls worked with Peak AI to streamline their bid process with contractors. This allows customers to get the answers they need in terms of bid decisions and quotes quickly and efficiently, significantly speeding up the sales cycle.

AI-as-a-Service is not a new concept. Canadian start-up Element AI tried to create an AI services business for non-tech companies to use as they might these days use consulting services. It never quite got there, though, and was acquired by ServiceNow last year. Peak AI is looking at specific elements such as sales, planning and supply chain for physical products in how decisions are made and where adding some level of automation in the decision is beneficial. The Peak AI solution, CODI (Connected Decision Intelligence) sits as a layer of intelligence that between the other systems, ingesting the data and aiding in its utilisation.
The added tool to create a data-ingestion layer for business decision-making is quite a trend right now. For example, IBM’s Causal Inference 360 Toolkit offers access to multiple tools that can move the decision-making processes from “best guess” to concrete answers based on data, aiding data scientists to apply and understand causal inference in their models.
Implications on Business Processes
The bigger problem is not the volume of data, but the interpretation of it.
Data warehouses and other ways of gathering data to a central or cloud-based location to digest is also not new. The real challenge lies with the interpretation of what the data means and what decisions can be fine-tuned with this data. This implies that data modelling and process engineers need to be involved. Not every company has thought through the possible options for their processes, nor are they necessarily ready to implement these new processes both in terms of resources and priorities. This also requires data harmonisation rules, consistent data quality and managed data operations.
Given the increasing flow of data in most organisations, external service providers for AI solution layers embedded in the infrastructure as data filters could be helpful in making sense of what exists. And they can perhaps suggest how the processes themselves can be readjusted to match the growth possibilities of the business itself. This is likely a great footprint for the likes of Accenture, KPMG and others as process wranglers.
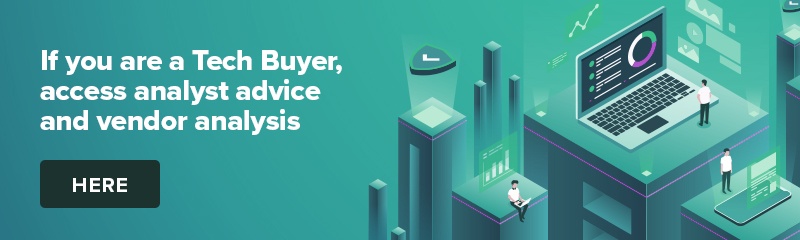
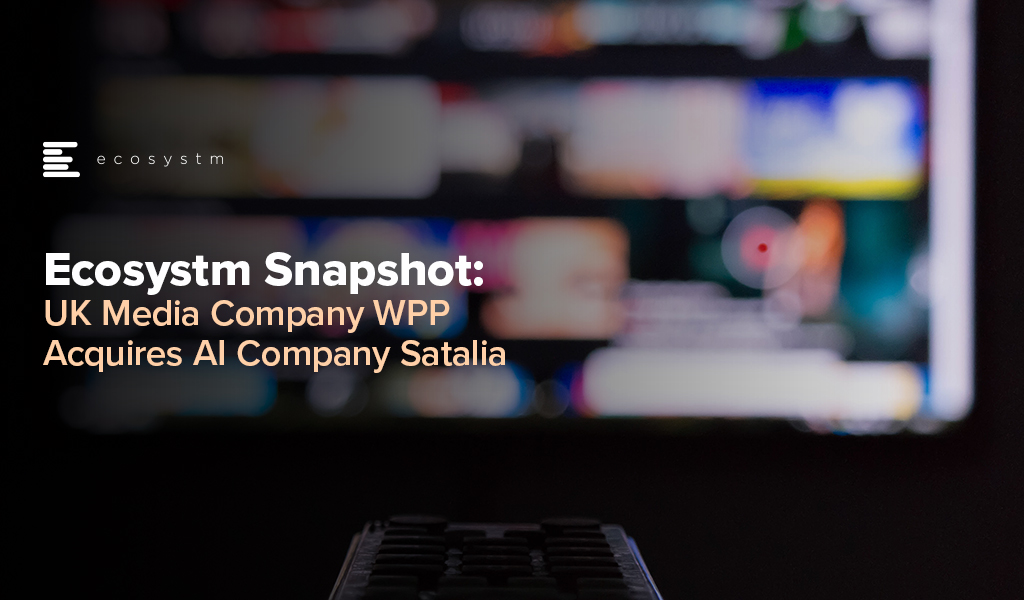
The process of developing advertising campaigns is evolving with the increasing use of artificial intelligence (AI). Advertisers want to optimise the amount of data at their disposal to craft better campaigns and drive more impact. Since early 2020, there has been a real push to integrate AI to help measure the effectiveness of campaigns and where to allocate ad spend. This now goes beyond media targeting and includes planning, analytics and creative. AI can assist in pattern matching, tailoring messages through AI-enabled hyper-personalisation, and analysing traffic to communicate through pattern identification of best times and means of communication. AI is being used to create ad copy; and social media and online advertising platforms are starting to roll out tools that help advertisers create better ads.
Ecosystm research shows that Media companies report optimisation, targeting and administrative functions such as billing are aided by AI use (Figure 1). However, the trend of Media companies leveraging AI for content design and media analysis is growing.
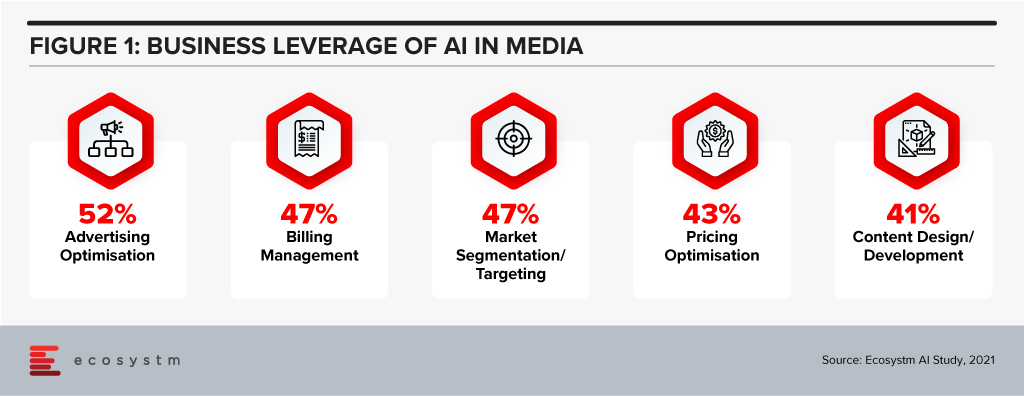
WPP Strengthening Tech Capabilities
This week, WPP announced the acquisition of Satalia, a UK-based company, who will consult with all WPP agencies globally to promote AI capabilities across the company and help shape the company’s AI strategy, including research and development, AI ethics, partnerships, talent and products.
It was announced that Satalia, whose clients include BT, DFS, DS Smith, PwC, Gigaclear, Tesco and Unilever, will join Wunderman Thompson Commerce to work on the technology division of their global eCommerce consultancy. Prior to the acquisition, Satalia had launched tools such as Satalia Workforce to automate work assignments; and Satalia Delivery, for automated delivery routes and schedules. The tools have been adopted by companies including PwC, DFS, Selecta and Australian supermarket chain Woolworths.
Like other global advertising organisations, WPP has been focused on expanding the experience, commerce and technology parts of the business, most recently acquiring Brazilian software engineering company DTI Digital in February. WPP also launched their own global data consultancy, Choreograph, in April. Choreograph is WPP’s newly formed global data products and technology company focused on helping brands activate new customer experiences by turning data into intelligence. This article from last year from the WPP CTO is an interesting read on their technology strategy, especially their move to cloud to enable their strategy.

Ethics & AI – The Right Focus
The acquisition of Satalia will give WPP and opportunity to evaluate important areas such as AI ethics, partnerships and talent which will be significantly important in the medium term. AI ethics in advertising is also a longer-term discussion. With AI and machine learning, the system learns patterns that help steer targeting towards audiences that are more likely to convert and identify the best places to get your message in front of these buyers. If done responsibly it should provide consumers with the ability to learn about and purchase relevant products and services. However, as we have recently discussed, AI has two main forms of bias – underrepresented data and developer bias – that also needs to be looked into.
Summary
The role of AI in the orchestration of the advertising process is developing rapidly. Media firms are adopting cloud platforms, making IP investments, and developing partnerships to build the support they can offer with their advertising services. The use of AI in advertising will help mature and season the process to be even more tailored to customer preferences.
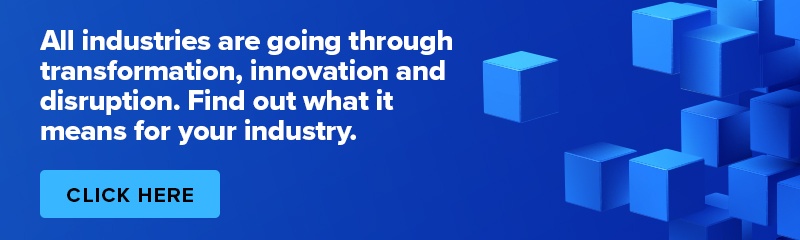
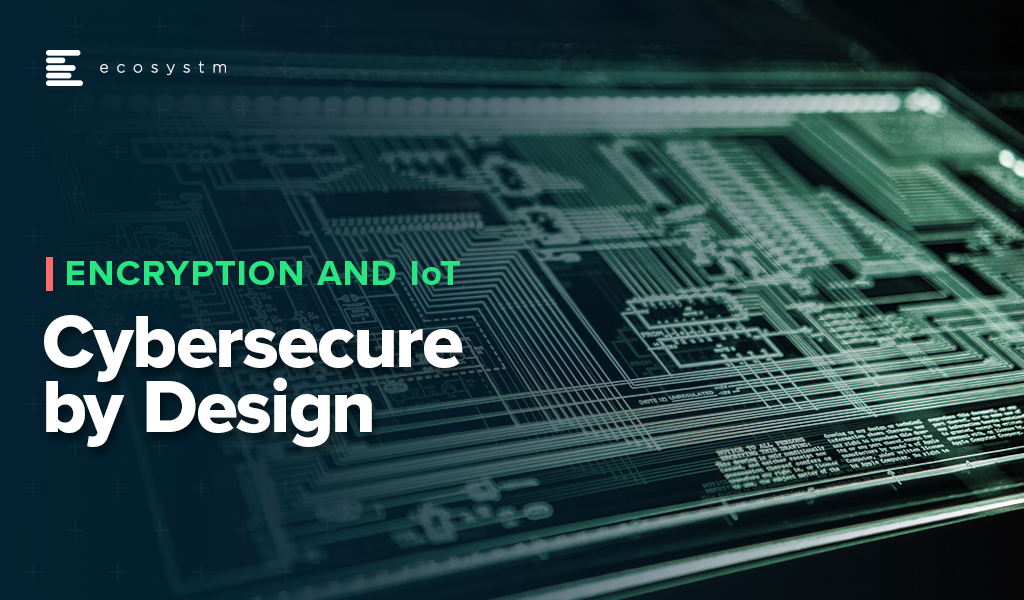
As we return to the office, there is a growing reliance on devices to tell us how safe and secure the environment is for our return. And in specific application areas, such as Healthcare and Manufacturing, IoT data is critical for decision-making. In some sectors such as Health and Wellness, IoT devices collect personally identifiable information (PII). IoT technology is so critical to our current infrastructures that the physical wellbeing of both individuals and organisations can be at risk.
Trust & Data
IoT are also vulnerable to breaches if not properly secured. And with a significant increase in cybersecurity events over the last year, the reliance on data from IoT is driving the need for better data integrity. Security features such as data integrity and device authentication can be accomplished through the use of digital certificates and these features need to be designed as part of the device prior to manufacturing. Because if you cannot trust either the IoT devices and their data, there is no point in collecting, running analytics, and executing decisions based on the information collected.
We discuss the role of embedding digital certificates into the IoT device at manufacture to enable better security and ongoing management of the device.
Securing IoT Data from the Edge
So much of what is happening on networks in terms of real-time data collection happens at the Edge. But because of the vast array of IoT devices connecting at the Edge, there has not been a way of baking trust into the manufacture of the devices. With a push to get the devices to market, many manufacturers historically have bypassed efforts on security. Devices have been added on the network at different times from different sources.
There is a need to verify the IoT devices and secure them, making sure to have an audit trail on what you are connecting to and communicating with.
So from a product design perspective, this leads us to several questions:
- How do we ensure the integrity of data from devices if we cannot authenticate them?
- How do we ensure that the operational systems being automated are controlled as intended?
- How do we authenticate the device on the network making the data request?
Using a Public Key Infrastructure (PKI) approach maintains assurance, integrity and confidentiality of data streams. PKI has become an important way to secure IoT device applications, and this needs to be built into the design of the device. Device authentication is also an important component, in addition to securing data streams. With good design and a PKI management that is up to the task you should be able to proceed with confidence in the data created at the Edge.
Johnson Controls/DigiCert have designed a new way of managing PKI certification for IoT devices through their partnership and integration of the DigiCert ONE™ PKI management platform and the Johnson Controls OpenBlue IoT device platform. Based on an advanced, container-based design, DigiCert ONE allows organisations to implement robust PKI deployment and management in any environment, roll out new services and manage users and devices across your organisation at any scale no matter the stage of their lifecycle. This creates an operational synergy within the Operational Technology (OT) and IoT spaces to ensure that hardware, software and communication remains trusted throughout the lifecycle.

Rationale on the Role of Certification in IoT Management
Digital certificates ensure the integrity of data and device communications through encryption and authentication, ensuring that transmitted data are genuine and have not been altered or tampered with. With government regulations worldwide mandating secure transit (and storage) of PII data, PKI can help ensure compliance with the regulations by securing the communication channel between the device and the gateway.
Connected IoT devices interact with each other through machine to machine (M2M) communication. Each of these billions of interactions will require authentication of device credentials for the endpoints to prove the device’s digital identity. In such scenarios, an identity management approach based on passwords or passcodes is not practical, and PKI digital certificates are by far the best option for IoT credential management today.
Creating lifecycle management for connected devices, including revocation of expired certificates, is another example where PKI can help to secure IoT devices. Having a robust management platform that enables device management, revocation and renewal of certificates is a critical component of a successful PKI. IoT devices will also need regular patches and upgrades to their firmware, with code signing being critical to ensure the integrity of the downloaded firmware – another example of the close linkage between the IoT world and the PKI world.
Summary
PKI certification benefits both people and processes. PKI enables identity assurance while digital certificates validate the identity of the connected device. Use of PKI for IoT is a necessary trend for sense of trust in the network and for quality control of device management.
Identifying the IoT device is critical in managing its lifespan and recognizing its legitimacy in the network. Building in the ability for PKI at the device’s manufacture is critical to enable the device for its lifetime. By recognizing a device, information on it can be maintained in an inventory and its lifecycle and replacement can be better managed. Once a certificate has been distributed and certified, having the control of PKI systems creates life-cycle management.
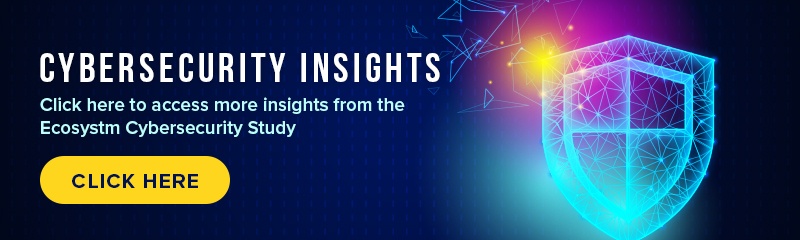