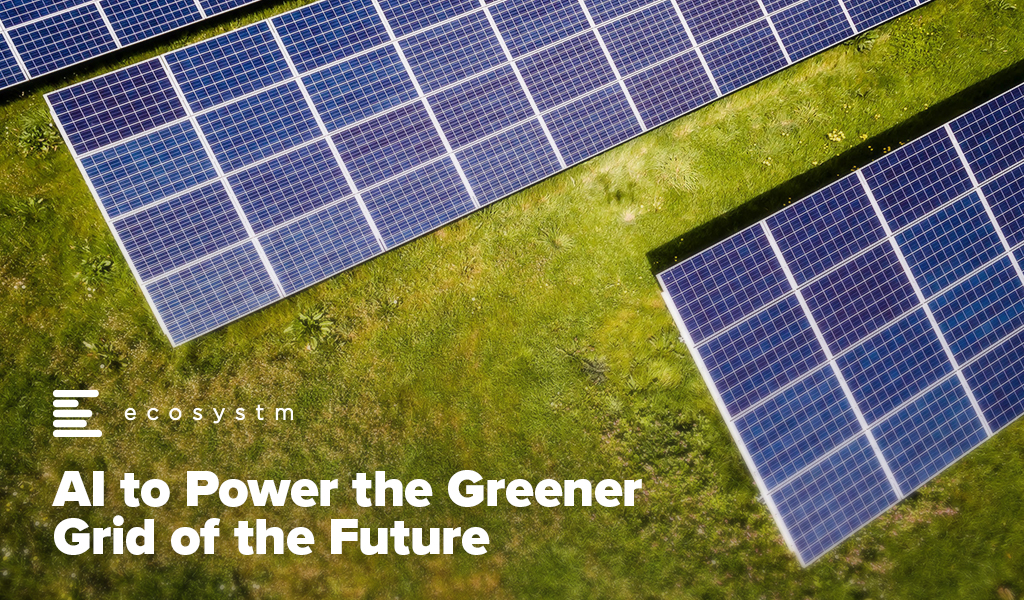
Energy providers around the world have transformed their electricity generation profiles to include solar, wind, hydro, and geothermal to reduce the carbon intensity of their economies. Many countries have surpassed expectations by approaching or exceeding 50% of production stemming from renewable sources. Concurrently, the decarbonisation of the transportation sector and the growing use of air conditioning is putting upward pressure on electricity demand.
Energy providers are keen on leveraging AI in several areas (Figure 1).
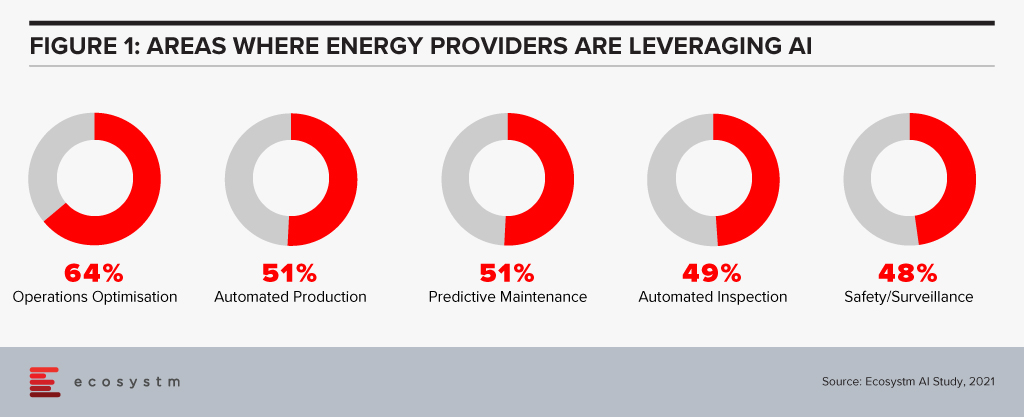
The sudden evolution of the Energy sector is creating new complexity in the grid, which human operators will be unable to monitor and manage without the assistance of AI.
Predicting Supply and Shaping Demand
Output Forecasting. The primary inhibitor to the mass adoption of renewable energy is the issue of intermittency. Solar is affected by shorter winter days and cloud cover, while wind turbines are ineffective during periods of low or even high wind speed. If electricity supply does not precisely match demand, grid operators must fire up costly and carbon-intensive peaker plants to fill the gap or resort to rolling blackouts. AI is becoming a tool for generators and grid operators to forecast renewable output more accurately, insight which can, in turn, be used to shape demand.
Competitive Pricing. Wind farm operators are beginning to use adversarial AI to judge if publicly available data for velocity, pressure, and density obey turbulent flow physics and if not, to refine their forecasts. Equipped with more accurate projections, they can bid with greater certainty on day-ahead auctions rather than relying on less profitable spot prices. This consequently makes wind power more competitive with fossil-fuel-based generators and smoothens out hourly price variability.
Predicting Usage Patterns. Improved temperature and humidity forecasts can also be used by grid operators to carry out peak shaving – encouraging consumers to reduce consumption during high-load periods. By accurately predicting intervals of increased use of home heating or cooling, programs such as Rush Hour Rewards by Nest, allow distributors to remotely adjust thermostats during seasonal extremes for cash incentives. Advanced knowledge of these weather events can also give grid operators the chance to temporarily lift regulatory hurdles or conduct emergency maintenance to ensure maximum capacity is available.
Supply Orchestration. Home battery systems and electric vehicles are growing in acceptance and their storage capacity will eventually become an important piece of infrastructure for time-shifting supply to match demand. The increasing build out of solar PV has created an oversupply in the middle of the day while the rising adoption of home air conditioning creates a spike in demand after working hours, resulting in the so-called Duck Curve (see Figure 2).
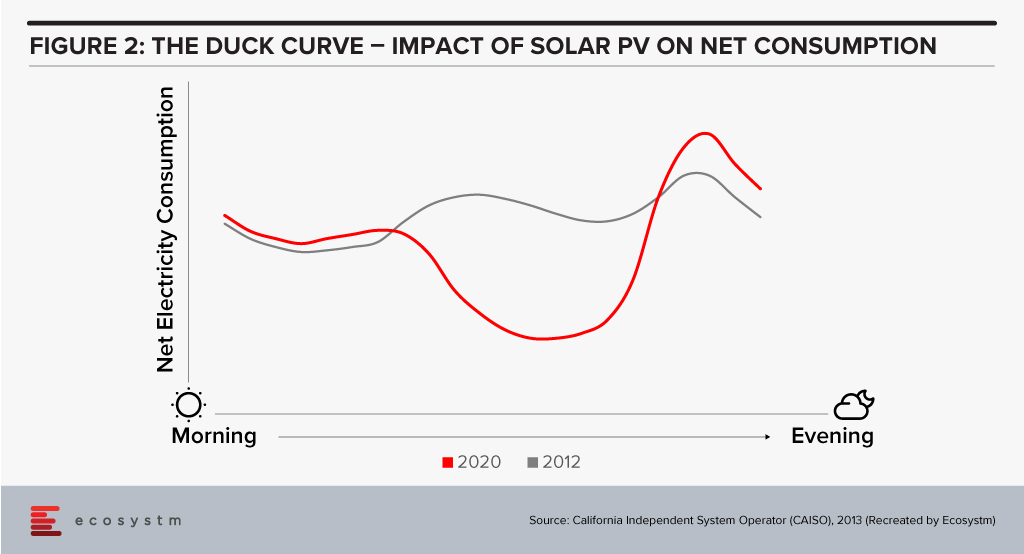
By predicting periods of potential supply shortfall, distributors can increase prices to a level attractive enough to prompt battery owners to sell excess electricity rather than store it. The complexity inherent in such a distributed system is only manageable with machine learning to constantly optimise pricing and supply orchestration to simultaneously prevent excessive degradation of battery performance. This is already available for large scale battery operators, e.g. using Tesla Autobidder, and will become accessible to networks of home and eventually vehicle owners.

The Future
Optimising Renewable Generation with AI
Renewable energy sources continue to make efficiency gains due to engineering improvements. However, advances in AI will increase generation even further. Solar PV and solar concentrators that rotate on dual-axis trackers to follow the path of the sun must each operate individually according to their own precise position and the time of day and year. This must be balanced for efficiency to reduce excessive movement, which consumes a portion of electricity output. Neural networks and fuzzy logic can be applied to optimise rotation to maximise production while reducing power consumption for operation. Input variables can include position, time, temperature, and even sky colour. Similarly, wind turbines can dynamically alter their positions to maximise wind flow across the entire fleet rather than at an individual level. The large streams of data must be processed in real-time as wind variables change to have an immediate effect on output.
Stabilising the Super Grid
To improve resiliency and lessen the effects of renewable intermittency, there is a growing push towards increasing the interconnectivity of national grids. This ensures supply even when regional generators go offline or if sudden local peaks in demand occur. Moreover, interconnected grids help even out supply from renewable sources using the philosophy that it is always windy or sunny somewhere. For example, the proposed European super grid would take advantage of higher wind generation in northern countries in winter and in North Africa in the summer. Additionally, hydroelectric plants in the north could be modified to become pumped storage facilities powered by solar thermal plants in the south to supply all of Europe.
Not only will a super grid require investment in new infrastructure, such as high voltage direct current (HVDC) for efficient long-distance transmission but also in intelligent systems to manage the new complexity. The retirement of fossil-fuel generators and greater variability of renewable sources will require rethinking grid inertia and frequency control between countries. Measurement solutions, such as GridMetrix by Reactive, have been deployed by AEMO in Australia and National Grid in the UK to better monitor how inertia fluctuates as renewable sources ebb and flow. Once real-time data becomes available for analysis, infrastructure such as synchronous condensers and quick-response batteries can be automatically utilised to regulate frequency.
A Positive Outlook
Countries such as China, India, the US, Germany, and Spain have shown that it is possible to add large amounts of solar and wind generation capacity at a pace. The next chapter in the renewable revolution will be ensuring that this can be done at scale without disrupting the grid and AI will be a key component in managing the transition.
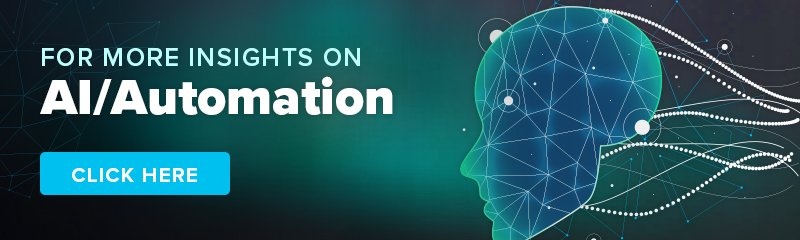