As economies around the world are beginning to recover from the recessions and slowdowns caused by the pandemic, we are beginning to witness, what I like to call, the “Great Bounce Forward”.
Why the Great Bounce Forward? Because too many businesses, journalists and economists are talking about businesses “bouncing back”. But there is no bounce back. We are bouncing into the “economic unknown”. The trading conditions we see today are nothing like what they were at the beginning of 2020. While many people refer to the “new normal” I have heard few talks about how they are or will benefit from these new market conditions.
Bouncing back may not be relevant as we negotiate the economic unknown – it is time to evaluate how we can bounce forward!
Leaping Ahead Through Digital First
Customer interactions have changed – digital-first is now a requirement – and many customers expect a personalised and optimised experience. Many companies are starting to personalise experiences today – thinking they are “delighting customers” through personalised transactions and journeys. But you don’t delight customers by giving them what they want – you disappoint them if you don’t offer a true personalised experience.
Digital changes are coming thick and fast. For example, Australia Post has announced that online sales are currently 20% higher than what they were at the previous highest peak in December 2020. Yes – much of Australia is in a lockdown, but online sales are dwarfing what they were during lockdowns in 2020.
But it is not just about offering online sales. In the digital world, customers now expect to be able to track packages, get alerts when they are delivered, and have access to easy and free returns. Again – if you don’t do this today, you are creating poor customer experiences and are most likely losing business to those that offer great experiences.
Here is what organisations are witnessing:
The need to evolve their CRM solution. Salespeople expect the CRM to give them insights on who to sell to, why to sell to them and what approach will work best. CRM systems that don’t provide this analysis are letting businesses and salespeople down.
Analytics has to be turned into actions. More businesses are telling their analytics partners to stop telling them what to do, and just do it! Automating the outcomes of BI and analytics is beginning to be expected.
Ease of use has become essential. Interactions and processes need to be intelligent and easy to automate. We no longer throw teams of people at challenges – we automate the outcomes and use technology to deliver entirely new experiences without teams of employees pulling strings behind the scenes.
Process and technology changes happen quickly and seamlessly. We have been taught this by Zoom, Microsoft, AWS and Google. If you aren’t doing this today, you are behind the market and behind the expectations of your employees and customers.
Ecosystems are emerging to enable this agility and innovation. We can now innovate with a growing range of partners. Companies can partner for a single sale and move on. Start-ups are being embraced by dinosaurs, and competitors are becoming partners. More companies than ever are involving their own customers in their innovation processes. Ecosystems are changing the ability of technology and business teams to offer new and improved services to customers and employees.

Time for a Shift in Organisational Culture
Seemingly, the world changed overnight. But many of these changes have been in the works for years. It just took a global crisis to highlight how important they are and how much organisations need to change to embrace these opportunities. The only thing holding businesses back from thriving in the Great Bounce Forward are their people and culture. If you can embrace these changes, your businesses will move forward and emerge as different companies to the ones that entered the pandemic in early 2020. You’ll be more open, agile, innovative and digitally aware. You’ll be able to move in new, unheralded directions, driving improved customer, shareholder, or citizen value.
So stop thinking about how your business will bounce back. Make plans for it to bounce forward into the unknown.
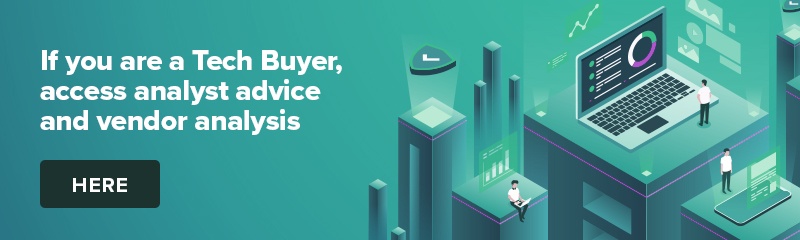
If you are a digital leader in the Financial Services industry (FSI), you have already heard this from your customers: ‘Why is it that Netflix and Amazon can make more relevant and personalised offers than my bank or wealth manager?’ Digital first players are obsessed with using data to understand their customer’s commercial and consumer behaviour. Financial Services will need to become just as obsessed with personalisation of offerings and services if they want to remain relevant to their customers. Ecosystm research finds that leveraging data to offer personalised service and product offerings to their clients is the leading digital priority in more than 50% of FSI organisations.
Banks, particularly, are both in a strong position and have a strong incentive to offer this personalisation. Their retail customers’ expectations are now shaped by the experience they have received from their favorite digital first firms, and they are making it increasingly clear that they expect personalised offerings from their banks. Furthermore, they are well positioned as a facilitator of commercial relationships between two segments of customers – consumers and merchants. The amount of data they hold on consumer interactions is comprehensive – and more importantly they are a trusted custodian of their customers’ data and privacy.
The Barriers to Personalisation
So, what is stopping them? Here are three insights from over 12 years of experience driving digitisation of Financial Services:
- Systems Legacy. Often the data and core banking systems do not allow for easy access and analysis of the required data across the data sets required (eg. Consumers and Merchants).
- Investment Priorities. There is still a significant investment happening in compliance and modernisation of core banking systems. Too often the focus of these programs can be myopic, and banks miss the opportunity to solve multiple pain points with their investments driven by overly focused problem statements.
- Culture and Purpose. Are banks stuck in a paradigm of their own making – defining their business models by what has served them well in the past? Will Amazon think about its provision of working capital to their small and medium business partners the same way as a bank does?
Vendor Focus – Crayon Data
Thankfully, there is a new breed of tech vendors who is making it easier for banks to drive personalisation of their offerings and connect customers from across segments. Crayon Data is a good example, with their maya.ai engine unearthing the preferences of customers and matching them to offerings from qualified merchants. It benefits all parties:
- The Consumer receives relevant offers, is served from discovery to fulfillment on a single platform and all personal data and information guarded by their bank.
- For Merchants, it allows them to reach the right customers at the right moment, develop valuable marketing and insights and all this directly from their bank partner’s platform.
- For Banks, it provides a scalable model for offer acquisition and easily configurable and measurable consumer engagement.
maya.ai leverages patented AI to create a powerful profile of each customer based on their buying habits and comparing these with millions of other consumers drawn in from their unstructured data sets and graph-based methodology. They then use their algorithms to assist their Financial Services client to make relevant offerings from qualified merchants to consumers in the right channel, at the right moment. All of this is done without exposing personal client information, as the data sets are based on behaviour rather than identity.

Conclusion
There are significant considerations for banks in offering these types of capabilities, such as:
- Privacy. While the technology operates on non-identifiable information, the perception of clients being ‘stalked’ by their bank in order to drive business to a merchant is one that would need to be managed carefully.
- Consumer opt-out. The ability for customers to opt out of this type of service is critical.
- Consumer financial wellbeing. It may be in the best interests of some consumer to not receive merchant offers, for instance where they are managing to a strict budget. These considerations can be baked into the overall customer journey (eg. prompts when the consumer is nearing their self-imposed monthly budget for a category), but care will need to be taken to keep customers’ best interests at heart.
While there are multiple challenges to overcome, the fact remains that personalisation is quickly becoming a core expectation for consumers. How will banks respond, and will we see AI use cases like Crayon Data become more prominent?
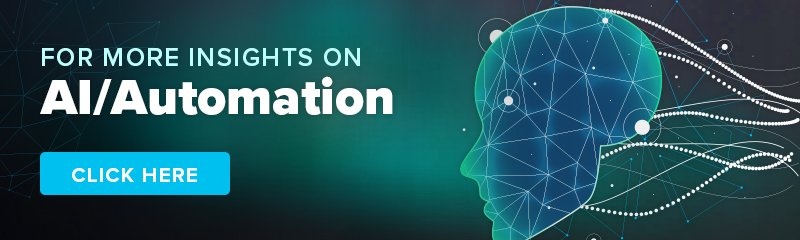
Last week I wrote about the need to remove hype from reality when it comes to AI. But what will ensure that your AI projects succeed?
It is quite obvious that success is determined by human aspects rather than technological factors. We have identified four key organisational actions that enable successful AI implementation at scale (Figure 1).
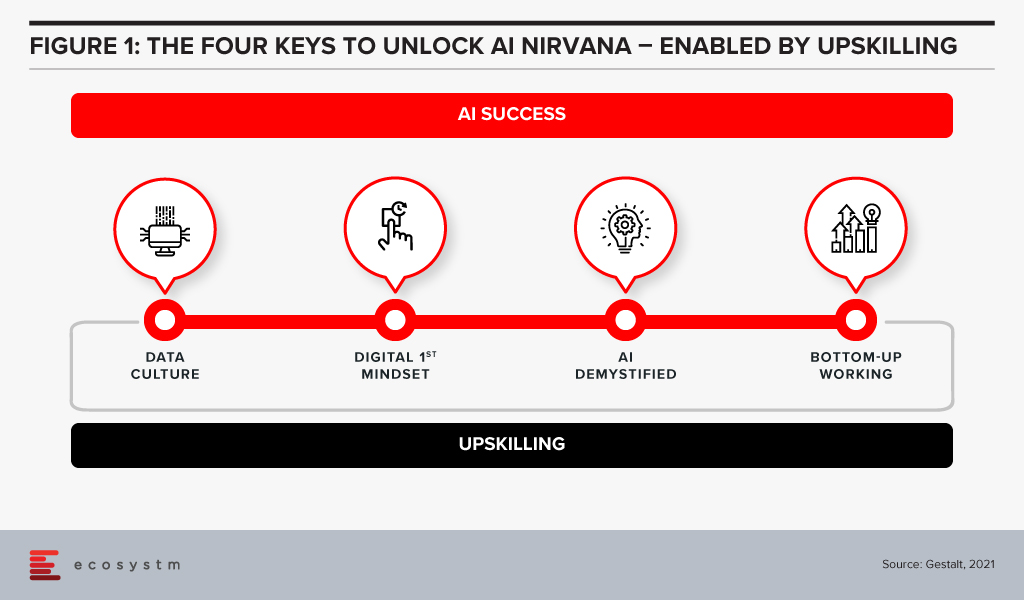
#1 Establish a Data Culture
The traditional focus for companies has been on ensuring access to good, clean data sets and the proper use of that data. Ecosystm research shows that only 28% of organisations focused on customer service, also focus on creating a data-driven organisational culture. But our experience has shown that culture is more critical than having the data. Does the organisation have a culture of using data to drive decisions? Does every level of the organisation understand and use data insights to do their day-to-day jobs? Is decision-making data-driven and decentralised, needing to be escalated only when there is ambiguity or need for strategic clarity? Do business teams push for new data sources when they are not able to get the insights they need?
Without this kind of culture, it may be possible to implement individual pieces of automation in a specific area or process, applying brute force to see it through. In order to transform the business and truly extract the power of AI, we advise organisations to build a culture of data-driven decision-making first. That organisational mindset, will make you capable implementing AI at scale. Focusing on changing the organisational culture will deliver greater returns than trying to implement piecemeal AI projects – even in the short to mid-term.
#2 Ingrain a Digital-First Mindset
Assuming a firm has passed the data culture hurdle, it needs to consider whether it has adopted a digital-first mindset. AI is one of many technologies that impact businesses, along with AR/VR, IoT, 5G, cloud and Blockchain to name a few. Today’s environment requires firms to be capable of utilising a variety of these technologies – often together – and possessing a workforce capable of using these digital tools.
A workforce with the digital-first mindset looks for a digital solution to problems wherever appropriate. They have a good understanding of digital technologies relevant to their space and understand key digital methodologies – such as Customer 360 to deliver a truly superior customer experience or Agile methodologies to successfully manage AI at scale.
AI needs business managers at the operational levels to work with IT or AI tech teams to pinpoint processes that are right for AI. They need to make an estimation based on historical data of what specific problems require an AI solution. This is enabled by the digital-first mindset.
#3 Demystify AI
The next step is to get business leaders, functional leaders, and business operational teams – not just those who work with AI – to acquire a basic understanding of AI.
They do not need to learn the intricacies of programming or how to create neural networks or anything nearly as technical in nature. However, all levels from the leadership down should have a solid understanding of what AI can do, the basics of how it works, how the process of training data results in improved outcomes and so on. They need to understand the continuous learning nature of AI solutions, getting better over time. While AI tools may recommend an answer, human insight is often needed to make a correct decision off this recommendation.

#4 Drive Implementation Bottom-Up
AI projects need alignment, objectives, strategy – and leadership and executive buy-in. But a very important aspect of an AI-driven organisation that is able to build scalable AI, is letting projects run bottom up.
As an example, a reputed Life Sciences company embarked on a multi-year AI project to improve productivity. They wanted to use NLP, Discovery, Cognitive Assist and ML to augment clinical proficiency of doctors and expected significant benefits in drug discovery and clinical trials by leveraging the immense dataset that was built over the last 20 years.
The company ran this like any other transformation project, with a central program management team taking the lead with the help of an AI Centre of Competency. These two teams developed a compelling business case, and identified initial pilots aligned with the long-term objectives of the program. However, after 18 months, they had very few tangible outcomes. Everyone including doctors, research scientists, technicians, and administrators, who participated in the program had their own interpretation of what AI was not able to do.
Discussion revealed that the doctors and researchers felt that they were training AI to replace themselves. Seeing a tool trying to mimic the same access and understanding of numerous documents baffled them at best. They were not ready to work with AI programs step-by-step to help AI tools learn and discover new insights.
At this point, we suggested approaching the project bottom-up – wherein the participating teams would decide specific projects to take up. This developed a culture where teams collaborated as well as competed with each other, to find new ways to use AI. Employees were shown a roadmap of how their jobs would be enhanced by offloading routine decisions to AI. They were shown that AI tools augment the employees’ cognitive capabilities and made them more effective.
The team working on critical trials found these tools extremely useful and were able to collaborate with other organisations specialising in similar trials. They created the metadata and used ML algorithms to discover new insights. Working bottom-up led to a very successful AI deployment.
We have seen time and again that while leadership may set the strategy and objectives, it is best to let the teams work bottom-up to come up with the projects to implement.
#5 Invest in Upskilling
The four “keys” are important to build an AI-powered, future-proof enterprise. They are all human related – and when they come together to work as a winning formula is when organisations invest in upskilling. Upskilling is the common glue and each factor requires specific kinds of upskilling (Figure 2).
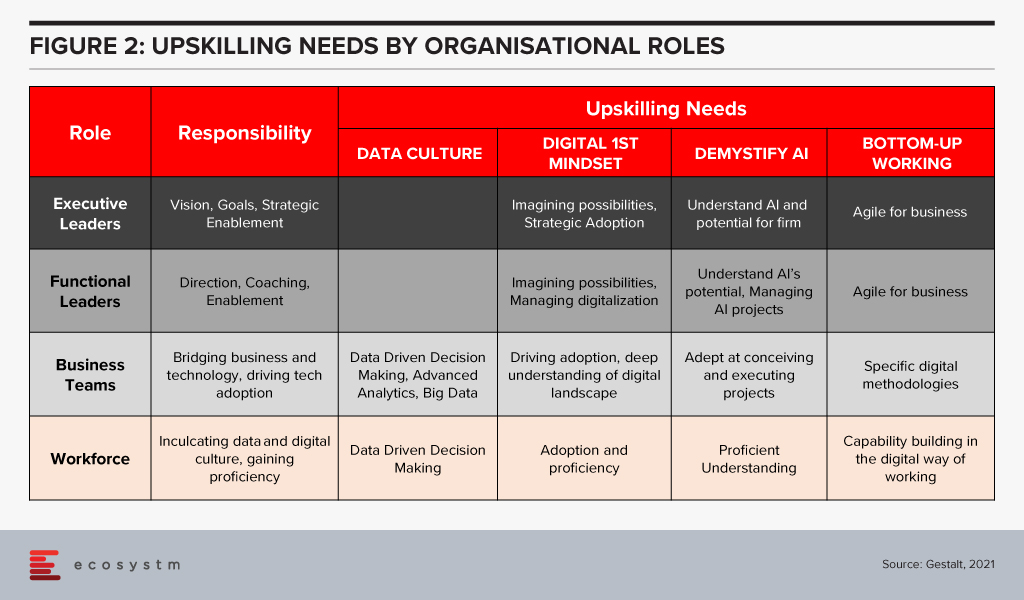
Upskilling needs vary by organisational level and the key being addressed. The bottom line is that upskilling is a universal requirement for driving AI at scale, successfully. And many organisations are realising it fast – Bosch and DBS Bank are some of the notable examples.
How much is your organisation invested in upskilling for AI implementation at scale? Share your stories in the comment box below.
Written with contributions from Ravi Pattamatta and Ratnesh Prasad
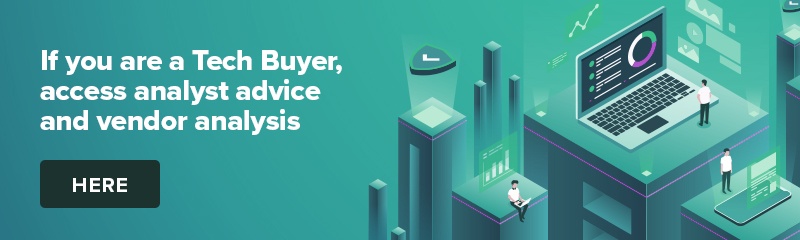