The Manufacturing industry is at crossroads today. It faces challenges such as geopolitical risks, supply chain disruptions, changing regulatory environments, workforce shortages, and changing consumer demands. Overcoming these requires innovation, collaboration, and proactive adaptation.
Fortunately, many of these challenges can be mitigated by technology. The future of Manufacturing will be shaped by advanced technology, automation, and AI. We are seeing early evidence of how smart factories, robotics, and 3D printing are transforming production processes for increased efficiency and customisation.
Manufacturing is all set to become more agile, efficient, and sustainable.
Read on to find out the changing priorities and key trends in Manufacturing; about the World Economic Forum’s Global Lighthouse Network initiative; and where Ecosystm advisor Kaushik Ghatak sees as the Future of Manufacturing.
Click here to download ‘The Future of Manufacturing’ as a PDF
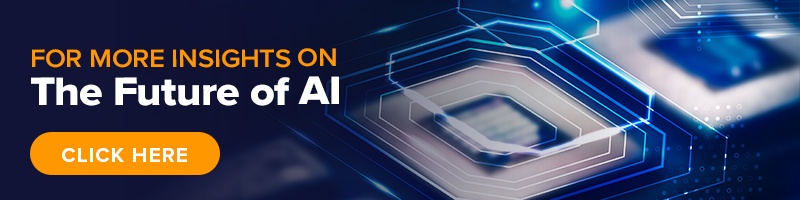
Energy providers around the world have transformed their electricity generation profiles to include solar, wind, hydro, and geothermal to reduce the carbon intensity of their economies. Many countries have surpassed expectations by approaching or exceeding 50% of production stemming from renewable sources. Concurrently, the decarbonisation of the transportation sector and the growing use of air conditioning is putting upward pressure on electricity demand.
Energy providers are keen on leveraging AI in several areas (Figure 1).
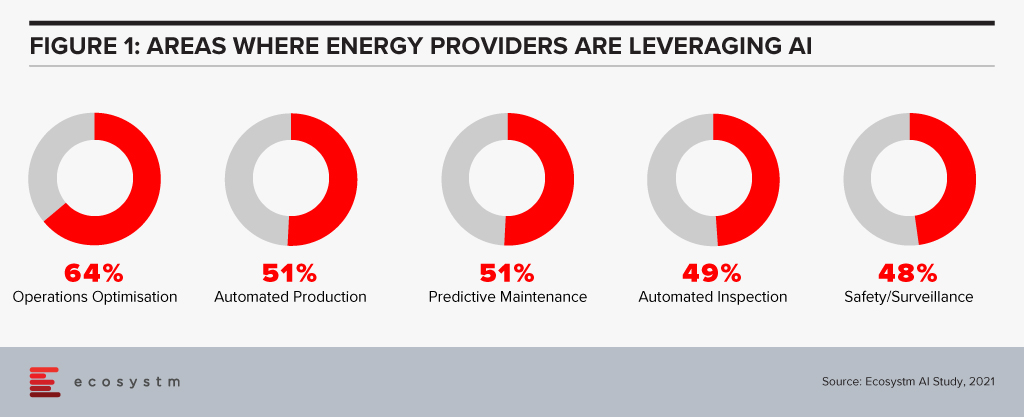
The sudden evolution of the Energy sector is creating new complexity in the grid, which human operators will be unable to monitor and manage without the assistance of AI.
Predicting Supply and Shaping Demand
Output Forecasting. The primary inhibitor to the mass adoption of renewable energy is the issue of intermittency. Solar is affected by shorter winter days and cloud cover, while wind turbines are ineffective during periods of low or even high wind speed. If electricity supply does not precisely match demand, grid operators must fire up costly and carbon-intensive peaker plants to fill the gap or resort to rolling blackouts. AI is becoming a tool for generators and grid operators to forecast renewable output more accurately, insight which can, in turn, be used to shape demand.
Competitive Pricing. Wind farm operators are beginning to use adversarial AI to judge if publicly available data for velocity, pressure, and density obey turbulent flow physics and if not, to refine their forecasts. Equipped with more accurate projections, they can bid with greater certainty on day-ahead auctions rather than relying on less profitable spot prices. This consequently makes wind power more competitive with fossil-fuel-based generators and smoothens out hourly price variability.
Predicting Usage Patterns. Improved temperature and humidity forecasts can also be used by grid operators to carry out peak shaving – encouraging consumers to reduce consumption during high-load periods. By accurately predicting intervals of increased use of home heating or cooling, programs such as Rush Hour Rewards by Nest, allow distributors to remotely adjust thermostats during seasonal extremes for cash incentives. Advanced knowledge of these weather events can also give grid operators the chance to temporarily lift regulatory hurdles or conduct emergency maintenance to ensure maximum capacity is available.
Supply Orchestration. Home battery systems and electric vehicles are growing in acceptance and their storage capacity will eventually become an important piece of infrastructure for time-shifting supply to match demand. The increasing build out of solar PV has created an oversupply in the middle of the day while the rising adoption of home air conditioning creates a spike in demand after working hours, resulting in the so-called Duck Curve (see Figure 2).
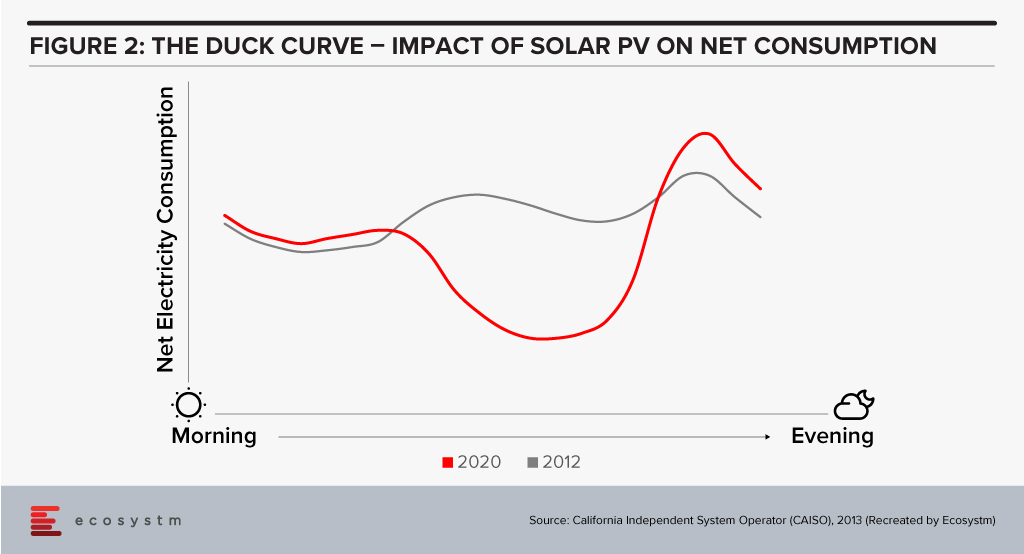
By predicting periods of potential supply shortfall, distributors can increase prices to a level attractive enough to prompt battery owners to sell excess electricity rather than store it. The complexity inherent in such a distributed system is only manageable with machine learning to constantly optimise pricing and supply orchestration to simultaneously prevent excessive degradation of battery performance. This is already available for large scale battery operators, e.g. using Tesla Autobidder, and will become accessible to networks of home and eventually vehicle owners.

The Future
Optimising Renewable Generation with AI
Renewable energy sources continue to make efficiency gains due to engineering improvements. However, advances in AI will increase generation even further. Solar PV and solar concentrators that rotate on dual-axis trackers to follow the path of the sun must each operate individually according to their own precise position and the time of day and year. This must be balanced for efficiency to reduce excessive movement, which consumes a portion of electricity output. Neural networks and fuzzy logic can be applied to optimise rotation to maximise production while reducing power consumption for operation. Input variables can include position, time, temperature, and even sky colour. Similarly, wind turbines can dynamically alter their positions to maximise wind flow across the entire fleet rather than at an individual level. The large streams of data must be processed in real-time as wind variables change to have an immediate effect on output.
Stabilising the Super Grid
To improve resiliency and lessen the effects of renewable intermittency, there is a growing push towards increasing the interconnectivity of national grids. This ensures supply even when regional generators go offline or if sudden local peaks in demand occur. Moreover, interconnected grids help even out supply from renewable sources using the philosophy that it is always windy or sunny somewhere. For example, the proposed European super grid would take advantage of higher wind generation in northern countries in winter and in North Africa in the summer. Additionally, hydroelectric plants in the north could be modified to become pumped storage facilities powered by solar thermal plants in the south to supply all of Europe.
Not only will a super grid require investment in new infrastructure, such as high voltage direct current (HVDC) for efficient long-distance transmission but also in intelligent systems to manage the new complexity. The retirement of fossil-fuel generators and greater variability of renewable sources will require rethinking grid inertia and frequency control between countries. Measurement solutions, such as GridMetrix by Reactive, have been deployed by AEMO in Australia and National Grid in the UK to better monitor how inertia fluctuates as renewable sources ebb and flow. Once real-time data becomes available for analysis, infrastructure such as synchronous condensers and quick-response batteries can be automatically utilised to regulate frequency.
A Positive Outlook
Countries such as China, India, the US, Germany, and Spain have shown that it is possible to add large amounts of solar and wind generation capacity at a pace. The next chapter in the renewable revolution will be ensuring that this can be done at scale without disrupting the grid and AI will be a key component in managing the transition.
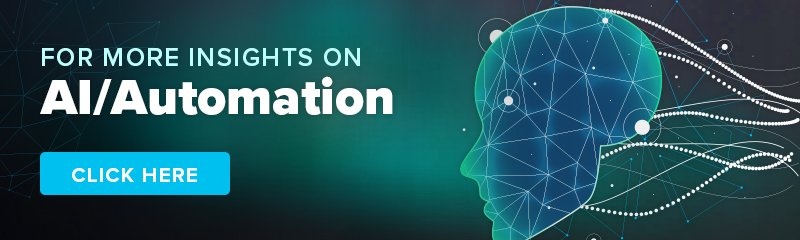
The rollout of 5G combined with edge computing in remote locations will change the way maintenance is carried out in the field. Historically, service teams performed maintenance either in a reactive fashion – fixing equipment when it broke – or using a preventative calendar-based approach. Neither of these methods is satisfactory, with the former being too late and resulting in failure while the latter is necessarily too early, resulting in excessive expenditure and downtime. The availability of connected sensors has allowed service teams to shift to condition monitoring without the need for taking equipment offline for inspections. The advent of analytics takes this approach further and has given us optimised scheduling in the form of predictive maintenance.
The next step is prescriptive maintenance in which AI can recommend action based on current and predicted condition according to expected usage or environmental circumstances. This could be as simple as alerting an operator to automatically ordering parts and scheduling multiple servicing tasks depending on forecasted production needs in the short term.
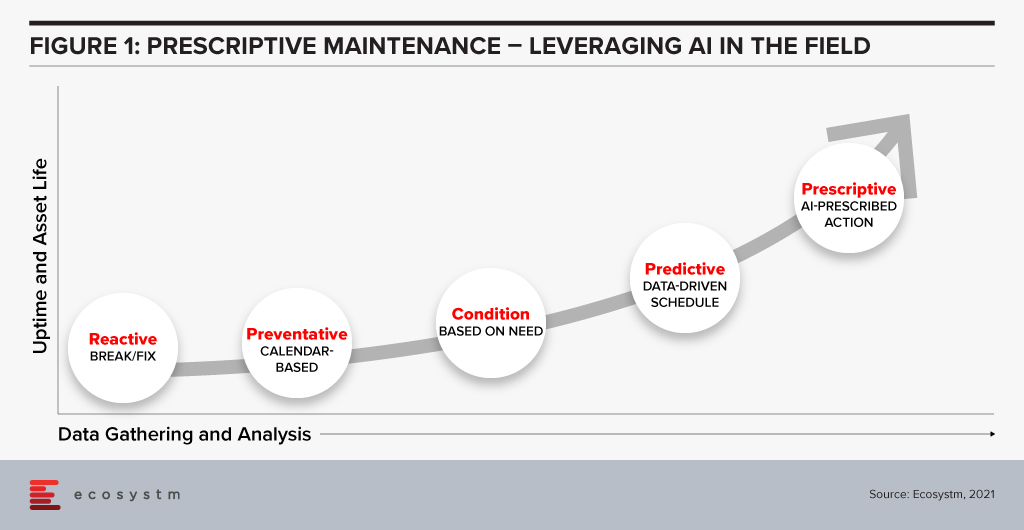
Prescriptive maintenance has only become possible with the advancement of AI and digital twin technology, but imminent improvements in connectivity and computing will take servicing to a new level. The rollout of 5G will give a boost to bandwidth, reduce latency, and increase the number of connections possible. Equipment in remote locations – such as transmission lines or machinery in resource industries – will benefit from the higher throughput of 5G connectivity, either as part of an operator’s network rollout or a private on-site deployment. Mobile machinery, particularly vehicles, which can include hundreds of sensors will no longer be required to wait until arrival before the condition can be assessed. Furthermore, vehicles equipped with external sensors can inspect stationary infrastructure as it passes by.
Edge computing – either carried out by miniature onboard devices or at smaller scale data centres embedded in 5G networks – ensure that intensive processing can be carried out closer to equipment than with a typical cloud environment. Bandwidth hungry applications, such as video and time series analysis, can be conducted with only meta data transmitted immediately and full archives uploaded with less urgency.
Prescriptive Maintenance with 5G and the Edge – Use Cases
- Transportation. Bridges built over railway lines equipped with high-speed cameras can monitor passing trains to inspect for damage. Data-intensive video analysis can be conducted on local devices for a rapid response while selected raw data can be uploaded to the cloud over 5G to improve inference models.
- Mining. Private 5G networks built-in remote sites can provide connectivity between fixed equipment, vehicles, drones, robotic dogs, workers, and remote operations centres. Autonomous haulage trucks can be monitored remotely and in the event of a breakdown, other vehicles can be automatically redirected to prevent dumping queues.
- Utilities. Emergency maintenance needs can be prioritised before extreme weather events based on meteorological forecasts and their impact on ageing parts. Machine learning can be used to understand location-specific effects of, for example, salt content in off-shore wind turbine cables. Early detection of turbine rotor cracks can recommend shutdown during high-load periods.

Data as an Asset
Effective prescriptive maintenance only becomes possible after the accumulation and integration of multiple data sources over an extended period. Inference models should understand both normal and abnormal equipment performance in various conditions, such as extreme weather, during incorrect operation, or when adjacent parts are degraded. For many smaller organisations or those deploying new equipment, the necessary volume of data will not be available without the assistance of equipment manufacturers. Moreover, even manufacturers will not have sufficient data on interaction with complementary equipment. This provides an opportunity for large operators to sell their own inference models as a new revenue stream. For example, an electrical grid operator in North America can partner with a similar, but smaller organisation in Europe to provide operational data and maintenance recommendations. Similarly, telecom providers, regional transportation providers, logistics companies, and smart cities will find industry players in other geographies that they do not naturally compete with.
Recommendations
- Employing multiple sensors. Baseline conditions and failure signatures are improved using machine learning based on feeds from multiple sensors, such as those that monitor vibration, sound, temperature, pressure, and humidity. The use of multiple sensors makes it possible to not only identify potential failure but also the reason for it and can therefore more accurately prescribe a solution to prevent an outage.
- Data assessment and integration. Prescriptive maintenance is most effective when multiple data sources are unified as inputs. Identify the location of these sources, such as ERP systems, time series on site, environmental data provided externally, or even in emails or on paper. A data fabric should be considered to ensure insights can be extracted from data no matter the environment it resides in.
- Automated action. Reduce the potential for human error or delay by automatically generating alerts and work orders for resource managers and service staff in the event of anomaly detection. Criticality measures should be adopted to help prioritise maintenance tasks and reduce alert noise.
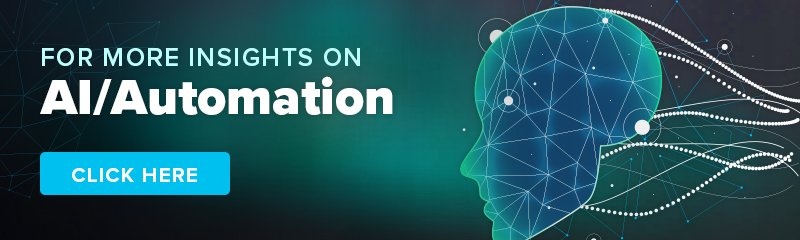
Microsoft announced in 2018 that they were investing US$ 5 billion globally in IoT innovation and research for the next 4 years – the focus being on secure IoT, creating development tools and intelligent services for IoT and the edge, and on growing their partner ecosystem.
Last year Microsoft’s industry updates showcased several IoT implementations across industries and their edge-based solutions portfolio, customers and partner ecosystem. The tech giant revealed nearly 150% YoY growth with customers such as Starbucks, Chevron, Walmart, Walgreens, BMW and Volkswagen added to the Azure platform, leveraging IoT services to accelerate their digital transformation journey. Microsoft also announced more than 70 partnerships with some of the big names in the IoT ecosystem, such as Universal Electronics, SAP, and Cradlepoint to extend solutions and support for the Microsoft IoT business.
Extending IoT Capabilities with Strategic Partnerships
There were several recent announcements which indicate that Microsoft is focused on strengthening their IoT and industry capabilities – and this is a timely move. Ecosystm Principal Advisor, Kaushik Ghatak says, “COVID-19 has brought to the fore the need for managing risks better. And the key to managing risks is to have better visibility and drive data-driven decisions; the sweet spot for IoT technologies. IoT is at the core of the Industry 4.0 story where deep domain expertise in industry verticals is a pre-requisite to success. It is heartening to see that Microsoft is taking the lead in building a powerful ecosystem by developing key partnerships with leading providers of Industry solutions.”
Last week, Microsoft and Hitachi announced a strategic alliance to accelerate the digital transformation of the Manufacturing and Logistics industries across Southeast Asia, Japan and North America. The first solutions are expected to be made available in Thailand as early as this month. Hitachi brings to the table their industry solutions, such as Lumada, and their IoT-ready industrial controllers HX Series. These solutions will be fully integrated with the Microsoft cloud platform, leveraging Azure, Dynamics 365 and Microsoft 365.
The three areas where the Hitachi solution is expected to bring strength to Microsoft’s industry offering are:
- Process optimisation and increased manufacturing productivity. Hitachi Digital Supply Chain and Azure IoT leveraged to analyse 4M data collected from manufacturing sites for visualisation/ analysis of production processes
- Logistics optimisation. Digital technologies such as Azure Maps and Hitachi Digital Solution for Logistics/Delivery Optimisation Service to analyse data on parameters such as traffic congestion, storage locations and delivery locations, to enabling smart routing
- Predictive maintenance and remote assist. HoloLens 2, Dynamics 365 Remote Assist and other smart devices, to empower first-line workers
Ecosystm Principal Advisor, Niloy Mukherjee feels that with projections of 43 – 100 billion IoT connected devices in the next few years, IoT is obviously a hot space. “We can think of IoT as a stack with four layers – the devices/sensors, the connection layer, the cloud and computing layer and the business apps layer. With Azure, Microsoft is very well positioned in the cloud and compute layer and can grab a large chunk of this fast-growing market. Tying with players like Hitachi allows Microsoft to integrate with the business apps layer and perhaps also some devices. It is absolutely the right strategy and I would expect them to go for many more such alliances. With Microsoft’s strength in the enterprise market, IoT gives them a great opportunity to increase their share of cloud workloads with customers.”
Addressing the Challenges of IoT Adoption
Ecosystm research shows that the biggest challenges in IoT adoption are security and integration concerns (Figure 1).
In 2018, when Microsoft started actively focusing on IoT, they also launched the Azure Certified for IoT program to maintain consistency and enhanced interoperability across their device partner ecosystem. This addresses the integration challenges that organisations face when deploying IoT. Microsoft continues to grow their IoT ecosystem, ensuring faster IoT deployments, with hardware and software that has been pre-tested and verified to work with Microsoft Azure IoT services. Last week also saw Cyient joining Microsoft Azure as a certified partner for IoT. Cyient IoT Edge Gateway 5400, their flagship IoT gateway product is now Microsoft Azure Certified for IoT. This is expected to accelerate IoT deployment for Cyient customers and enable a seamless integration of edge devices to the cloud.
Ghatak says, “To scale up their IoT business, Microsoft would need to develop a substantially large ecosystem, beyond few key players such as Hitachi, who dominate at the large enterprise segment of the market. That is where partnerships with smaller and niche industry solutions providers such as Cyient fits in. More niche providers such as Cyient will increase Microsoft’s reach into medium and smaller segments of the enterprise market.”
Addressing the Increasing Threat Landscape
Recent cyber-attack trends and security breach statistics reveal a huge increase in cybercrime activities, in the wake of the COVID-19 pandemic. As the number of IoT sensors, devices and gateways increase, so does the risk of security breaches. As shown in figure 1, cybersecurity concerns are real and can act as a barrier to IoT adoption, despite the benefits that the technology brings. Automated vulnerability management capabilities, that allow risk assessment and patch installation where necessary will see an increase in IoT adoption.
To complement Microsoft Azure IoT security, Microsoft acquired IoT security specialist CyberX, last month. The acquisition will enable greater security for the IoT devices connected to the Microsoft network and will help their customers to gain visibility through a map of devices thus allowing them to gather information on security risks associated with thousands of sensors and connected devices. This will enhance smart grid, smart manufacturing and digital assets and profiles and reduce vulnerabilities across production and supply chain.
Mukherjee says, “The key concern for the expansion of IoT into more and more use cases in the next few years is really going to be security. New areas like VR and AR are emerging from futuristic fantasy to real-world reality. These will tempt many enterprises – but security will be the key concern to address. And so, Microsoft’s simultaneous push on security completely aligns with this. As the Ecosystm MSSP VendorScope results show Microsoft’s strategy on cybersecurity seems to be working.”
Talking about Microsoft’s go-to-market strategy, Mukherjee adds, “Microsoft is obviously spreading its net far and wide for all cloud applications including IoT, to go-to-market with partners. One of the key focus area here is the SME segment, which is forecast to be one of the hot growing segments for IoT in the next few years. The more offerings from the business apps layer that Microsoft integrates, the more they enable their partners to sell to their customers.”
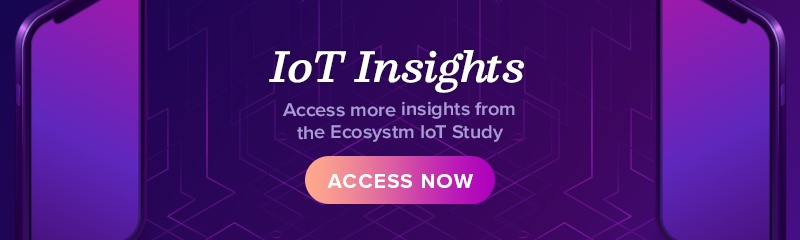
Authored by Ullrich Loeffler and Kaushik Ghatak
Technology has been identified as a key enabler of innovation and transformation with great potential to disrupt and reshape entire industries. At the same time, the technology industry itself has been at the forefront of disruption with thousands of promising start-ups emerging in areas such as artificial intelligence (AI), Blockchain, cloud and cybersecurity to name a few.
In February 2020, Ecosystm had the opportunity to meet the executive team of Avanseus, an AI solution provider that promises to reshape traditional maintenance processes by leveraging AI-based algorithms for predictive maintenance and failure detection.
Avanseus is not the newest kid on the block having been launched in June 2015 with headquarters in Singapore. The founding team has extensive experience in the telecommunications sector from executive careers in both the operator and the network provider segments. The problem statement and value proposition that Avanseus was founded on is to support telecom companies to tackle the costly challenge of maintaining their increasingly complex networks and to ensure network performance and customer satisfaction. Ecosystm research finds that predictive maintenance is a key priority for telecom providers looking to invest in newer technologies (Figure 1).
The solution Avanseus offers aims to be simple in the way that its proprietary AI-enabled algorithms can assess and predict network performance and network failures with as little as 6 months of network data history to achieve a high degree of prediction accuracy across telecom networks. The simplicity of the solution further allows cost-effective proofs of concept (PoCs) which lets telecom prospects experience first-hand its potential to drive down maintenance cost and ensure network performance.
Avanseus had secured seed capital of US$2.5 million till the middle of 2017. At the end of 2018, Avanseus secured funding via convertible notes of US$1.3m – main noteholders being TNB Spring and SEEDS Capital (Enterprise Singapore). A global network equipment manufacturer and managed services provider became its first commercial customer in 2016. From there Avanseus has demonstrated steady growth achieving revenue of US$1 million in 2018 and US$2.3 million in 2019. 2020 is forecast to be a milestone year with predictions to become cash-flow positive and to achieve revenue growth of 150% over 2019.
As of February 2020, Avanseus employs 41 staff across multiple international locations including its headquarters in Singapore, its development centre in Bangalore and sales offices in Delhi, North and Latin America and Italy to grow its global presence. The team is complemented by 8 part-time consultants and a growing partner ecosystem which includes major consulting firms as well as technology partners such as Dell, Splunk and Siemens. Expanding its partnerships globally is a key part of its strategy in order to scale up on the opportunities it can contest.
Avanseus’ Potential Growth Path
Considering its young history, Avanseus has shown an impressive growth path which can be credited to staying true to its game plan and its original value proposition and solution design. A new fund-raising round had been kicked off at the end of 2019 with the aim to secure sufficient capital to accelerate growth over the coming years. Half of the anticipated funding will be invested into on-site consultants and sales teams while the other half will be invested in R&D to expand automation into APIs and other machine learning technologies. R&D has been a key focus from its early days which has led to the filing of 8 patents, 2 of which have been granted.
In order to accelerate growth further, Avanseus is also re-assessing the industries that could benefit from its predictive maintenance solutions. As with many startups and growth companies, innovation is often not a straight path and new opportunities and ideas arise as the market and customers are engaged. Several industries face similar challenges and benefit from reduced maintenance cost, reduced downtime, extended equipment lifecycles and improved services quality. To transfer the value proposition across use cases and industry applications Avanseus is looking to leverage approximately 80% of its existing solution and apply 20% of industry-specific domain expertise. This has opened up new growth opportunities in a number of areas such as Industrial IoT, Utilities, Manufacturing and supply chain. There are also opportunities in customer-focused industries such as Banking in niche areas such as maintenance of data centre operations.
Ecosystm Comments
As companies collect and manage an exploding amount of data assets within their operations or from their customers, there is an increasing opportunity for innovative technology vendors to support these companies in driving value from their data assets. Avanseus has demonstrated a clear vision and execution in addressing one of these opportunities by focusing on a clear problem statement and offering a ‘simple’ solution that presents a strong business case. As with every growth company, the challenge is to leverage this opportunity and secure the right funding and resources to scale up as quickly as possible.
Partnerships will be critical in its growth path but signing up partners alone may not translate to creating value. The challenge for Avanseus will be to achieve partner commitment and enablement across different geographies. This will require time and a dedicated channel strategy beyond opportunistic partnerships that are born out of specific client engagements.
Another opportunity that could turn into a challenge is the new range of solution applications that Avanseus has identified. Being a high growth company, the greater challenge is often to decide what not to do rather than what can be done. Avanseus is well advised to carefully select which industries it wants to expand into and focus on. Each new solution set will present a magnet for additional resources and funding and may well be a distraction.